Pfam ID | Pfam Name | Expression Trials | Positive Outcomes | Negative Outcomes | AUC (95% CI) |
---|---|---|---|---|---|
PF00359.19 | PTS_EIIA_2 | 14 | 3 | 11 | 1.00 (1.00-1.00) |
PF02656.12 | DUF202 | 10 | 9 | 1 | 1.00 (1.00-1.00) |
PF03186.10 | CobD_Cbib | 11 | 1 | 10 | 1.00 (1.00-1.00) |
PF03176.12 | MMPL | 105 | 1 | 104 | 0.99 (0.99-0.99) |
PF02683.12 | DsbD | 18 | 1 | 17 | 0.97 (0.97-0.97) |
PF02355.13 | SecD_SecF | 33 | 1 | 32 | 0.97 (0.97-0.97) |
PF12822.4 | DUF3816 | 11 | 4 | 7 | 0.96 (0.87-1.00) |
PF00662.17 | Proton_antipo_N | 120 | 2 | 118 | 0.94 (0.87-1.00) |
PF04247.9 | SirB | 16 | 1 | 15 | 0.93 (0.93-0.93) |
PF06127.8 | DUF962 | 11 | 6 | 5 | 0.93 (0.78-1.00) |
PF11742.5 | DUF3302 | 15 | 1 | 14 | 0.93 (0.93-0.93) |
PF01610.14 | DDE_Tnp_ISL3 | 14 | 2 | 12 | 0.92 (0.73-1.00) |
PF07290.8 | DUF1449 | 11 | 1 | 10 | 0.90 (0.90-0.90) |
PF02659.12 | Mntp | 29 | 2 | 27 | 0.89 (0.76-1.00) |
PF04217.10 | DUF412 | 12 | 5 | 7 | 0.89 (0.65-1.00) |
PF05425.10 | CopD | 20 | 3 | 17 | 0.88 (0.73-1.00) |
PF02535.19 | Zip | 34 | 1 | 33 | 0.86 (0.86-0.86) |
PF02667.11 | SCFA_trans | 19 | 7 | 12 | 0.86 (0.68-1.00) |
PF01810.15 | LysE | 41 | 2 | 39 | 0.83 (0.59-1.00) |
PF06930.9 | DUF1282 | 12 | 5 | 7 | 0.83 (0.57-1.00) |
PF03595.14 | SLAC1 | 76 | 19 | 57 | 0.81 (0.67-0.94) |
PF04172.13 | LrgB | 63 | 4 | 59 | 0.79 (0.57-1.00) |
PF03601.11 | Cons_hypoth698 | 52 | 9 | 43 | 0.78 (0.64-0.92) |
PF02537.12 | CRCB | 81 | 20 | 61 | 0.77 (0.66-0.88) |
PF01757.19 | Acyl_transf_3 | 23 | 6 | 17 | 0.76 (0.53-1.00) |
PF02378.15 | PTS_EIIC | 82 | 15 | 67 | 0.76 (0.62-0.90) |
PF03929.13 | PepSY_TM | 25 | 2 | 23 | 0.76 (0.36-1.00) |
PF00999.18 | Na_H_Exchanger | 398 | 21 | 377 | 0.76 (0.66-0.86) |
PF00535.23 | Glycos_transf_2 | 282 | 62 | 220 | 0.76 (0.70-0.82) |
PF03605.11 | DcuA_DcuB | 28 | 2 | 26 | 0.75 (0.32-1.00) |
PF02690.12 | Na_Pi_cotrans | 64 | 9 | 55 | 0.75 (0.54-0.95) |
PF01699.21 | Na_Ca_ex | 75 | 4 | 71 | 0.74 (0.58-0.90) |
PF03390.12 | 2HCT | 18 | 3 | 15 | 0.73 (0.37-1.00) |
PF00950.14 | ABC-3 | 33 | 7 | 26 | 0.73 (0.51-0.94) |
PF06762.11 | LMF1 | 17 | 1 | 16 | 0.72 (0.72-0.72) |
PF03741.13 | TerC | 19 | 4 | 15 | 0.72 (0.42-1.00) |
PF02632.11 | BioY | 47 | 15 | 32 | 0.71 (0.54-0.88) |
PF06738.9 | ThrE | 26 | 2 | 24 | 0.71 (0.42-1.00) |
PF11911.5 | DUF3429 | 36 | 12 | 24 | 0.70 (0.53-0.88) |
PF01226.14 | Form_Nir_trans | 13 | 8 | 5 | 0.70 (0.38-1.00) |
PF09586.7 | YfhO | 6 | 1 | 5 | 0.70 (0.70-0.70) |
PF03739.11 | YjgP_YjgQ | 214 | 17 | 197 | 0.70 (0.56-0.84) |
PF05656.11 | DUF805 | 100 | 21 | 79 | 0.69 (0.56-0.81) |
PF01566.15 | Nramp | 129 | 21 | 108 | 0.69 (0.55-0.82) |
PF05977.10 | MFS_3 | 12 | 3 | 9 | 0.69 (0.37-1.00) |
PF03023.11 | MVIN | 67 | 7 | 60 | 0.68 (0.46-0.90) |
PF02674.13 | Colicin_V | 32 | 4 | 28 | 0.68 (0.47-0.89) |
PF03009.14 | GDPD | 46 | 1 | 45 | 0.68 (0.68-0.68) |
PF10110.6 | GPDPase_memb | 46 | 1 | 45 | 0.68 (0.68-0.68) |
PF01545.18 | Cation_efflux | 21 | 5 | 16 | 0.68 (0.32-1.00) |
PF07854.9 | DUF1646 | 7 | 1 | 6 | 0.67 (0.67-0.67) |
PF01594.13 | UPF0118 | 436 | 137 | 299 | 0.67 (0.61-0.72) |
PF06541.8 | ABC_trans_CmpB | 33 | 2 | 31 | 0.66 (0.51-0.81) |
PF12698.4 | ABC2_membrane_3 | 248 | 13 | 235 | 0.65 (0.46-0.85) |
PF01578.17 | Cytochrom_C_asm | 53 | 5 | 48 | 0.65 (0.39-0.92) |
PF00520.28 | Ion_trans | 17 | 4 | 13 | 0.65 (0.35-0.95) |
PF00230.17 | MIP | 83 | 35 | 48 | 0.65 (0.53-0.77) |
PF04608.10 | PgpA | 31 | 11 | 20 | 0.65 (0.43-0.87) |
PF03062.16 | MBOAT | 78 | 5 | 73 | 0.65 (0.36-0.94) |
PF01569.18 | PAP2 | 8 | 1 | 7 | 0.64 (0.64-0.64) |
PF03788.11 | LrgA | 55 | 3 | 52 | 0.64 (0.36-0.92) |
PF03824.13 | NicO | 15 | 3 | 12 | 0.64 (0.26-1.00) |
PF03222.10 | Trp_Tyr_perm | 13 | 5 | 8 | 0.64 (0.35-0.92) |
PF01040.15 | UbiA | 212 | 29 | 183 | 0.63 (0.53-0.73) |
PF02517.13 | Abi | 47 | 13 | 34 | 0.63 (0.43-0.82) |
PF07690.13 | MFS_1 | 378 | 45 | 333 | 0.62 (0.54-0.71) |
PF00854.18 | PTR2 | 103 | 17 | 86 | 0.62 (0.49-0.76) |
PF01627.20 | Hpt | 14 | 3 | 11 | 0.62 (0.30-0.94) |
PF00361.17 | Proton_antipo_M | 648 | 19 | 629 | 0.62 (0.51-0.72) |
PF02592.12 | Vut_1 | 34 | 7 | 27 | 0.61 (0.39-0.84) |
PF01758.13 | SBF | 126 | 22 | 104 | 0.61 (0.48-0.74) |
PF00860.17 | Xan_ur_permease | 559 | 115 | 444 | 0.61 (0.55-0.67) |
PF02702.14 | KdpD | 24 | 3 | 21 | 0.61 (0.22-1.00) |
PF13231.3 | PMT_2 | 111 | 8 | 103 | 0.61 (0.43-0.78) |
PF00474.14 | SSF | 124 | 9 | 115 | 0.60 (0.45-0.76) |
PF00916.17 | Sulfate_transp | 194 | 41 | 153 | 0.60 (0.50-0.70) |
PF12821.4 | ThrE_2 | 6 | 1 | 5 | 0.60 (0.60-0.60) |
PF03631.12 | Virul_fac_BrkB | 160 | 61 | 99 | 0.60 (0.51-0.69) |
PF05661.9 | DUF808 | 58 | 20 | 38 | 0.60 (0.45-0.74) |
PF03600.13 | CitMHS | 71 | 9 | 62 | 0.60 (0.37-0.82) |
PF07786.9 | DUF1624 | 42 | 10 | 32 | 0.60 (0.39-0.80) |
PF02447.13 | GntP_permease | 139 | 12 | 127 | 0.59 (0.44-0.75) |
PF01914.14 | MarC | 77 | 6 | 71 | 0.59 (0.37-0.81) |
PF01384.17 | PHO4 | 178 | 11 | 167 | 0.59 (0.39-0.79) |
PF03594.10 | BenE | 13 | 2 | 11 | 0.59 (0.37-0.81) |
PF03073.12 | TspO_MBR | 100 | 29 | 71 | 0.59 (0.48-0.70) |
PF04241.12 | DUF423 | 116 | 43 | 73 | 0.59 (0.48-0.70) |
PF09335.8 | SNARE_assoc | 429 | 51 | 378 | 0.59 (0.51-0.67) |
PF01554.15 | MatE | 659 | 101 | 558 | 0.59 (0.53-0.64) |
PF02233.13 | PNTB | 175 | 29 | 146 | 0.58 (0.46-0.71) |
PF01618.13 | MotA_ExbB | 32 | 14 | 18 | 0.58 (0.36-0.81) |
PF17113.2 | AmpE | 7 | 3 | 4 | 0.58 (0.07-1.00) |
PF01061.21 | ABC2_membrane | 328 | 48 | 280 | 0.58 (0.51-0.65) |
PF01292.17 | Ni_hydr_CYTB | 131 | 58 | 73 | 0.57 (0.47-0.67) |
PF05232.9 | BTP | 8 | 1 | 7 | 0.57 (0.57-0.57) |
PF01066.18 | CDP-OH_P_transf | 436 | 124 | 312 | 0.57 (0.51-0.63) |
PF00672.22 | HAMP | 130 | 40 | 90 | 0.57 (0.46-0.67) |
PF00892.17 | EamA | 813 | 125 | 688 | 0.57 (0.51-0.62) |
PF07681.9 | DoxX | 108 | 47 | 61 | 0.56 (0.45-0.67) |
PF03547.15 | Mem_trans | 292 | 18 | 274 | 0.56 (0.40-0.71) |
PF01148.17 | CTP_transf_1 | 161 | 38 | 123 | 0.56 (0.45-0.66) |
PF03006.17 | HlyIII | 93 | 7 | 86 | 0.55 (0.35-0.76) |
PF00939.16 | Na_sulph_symp | 109 | 19 | 90 | 0.55 (0.43-0.68) |
PF01169.16 | UPF0016 | 92 | 3 | 89 | 0.54 (0.16-0.91) |
PF02694.12 | UPF0060 | 21 | 2 | 19 | 0.53 (0.30-0.76) |
PF00083.21 | Sugar_tr | 285 | 46 | 239 | 0.52 (0.43-0.61) |
PF01434.15 | Peptidase_M41 | 51 | 7 | 44 | 0.52 (0.34-0.70) |
PF02719.12 | Polysacc_synt_2 | 62 | 19 | 43 | 0.52 (0.35-0.69) |
PF07947.11 | YhhN | 64 | 10 | 54 | 0.52 (0.37-0.67) |
PF01940.13 | DUF92 | 66 | 3 | 63 | 0.52 (0.26-0.77) |
PF00893.16 | Multi_Drug_Res | 160 | 7 | 153 | 0.52 (0.35-0.68) |
PF02628.12 | COX15-CtaA | 133 | 50 | 83 | 0.52 (0.42-0.61) |
PF00507.16 | Oxidored_q4 | 159 | 4 | 155 | 0.51 (0.18-0.85) |
PF06580.10 | His_kinase | 49 | 9 | 40 | 0.51 (0.28-0.74) |
PF02040.12 | ArsB | 26 | 7 | 19 | 0.50 (0.27-0.74) |
PF01553.18 | Acyltransferase | 20 | 4 | 16 | 0.50 (0.12-0.88) |
PF03253.11 | UT | 9 | 2 | 7 | 0.50 (0.00-1.00) |
PF06168.8 | DUF981 | 6 | 2 | 4 | 0.50 (0.00-1.00) |
PF09997.6 | DUF2238 | 13 | 5 | 8 | 0.50 (0.16-0.84) |
PF04304.10 | DUF454 | 69 | 14 | 55 | 0.49 (0.30-0.68) |
PF06271.9 | RDD | 84 | 20 | 64 | 0.49 (0.33-0.66) |
PF14667.3 | Polysacc_synt_C | 181 | 8 | 173 | 0.49 (0.35-0.64) |
PF06480.12 | FtsH_ext | 42 | 6 | 36 | 0.49 (0.32-0.66) |
PF02096.17 | 60KD_IMP | 256 | 43 | 213 | 0.49 (0.40-0.57) |
PF03806.10 | ABG_transport | 29 | 8 | 21 | 0.49 (0.25-0.72) |
PF07664.9 | FeoB_C | 103 | 15 | 88 | 0.49 (0.28-0.69) |
PF09515.7 | Thia_YuaJ | 16 | 6 | 10 | 0.48 (0.12-0.85) |
PF01595.17 | DUF21 | 238 | 50 | 188 | 0.48 (0.39-0.57) |
PF01943.14 | Polysacc_synt | 189 | 8 | 181 | 0.47 (0.28-0.66) |
PF02133.12 | Transp_cyt_pur | 21 | 4 | 17 | 0.46 (0.11-0.80) |
PF03606.12 | DcuC | 37 | 4 | 33 | 0.45 (0.05-0.86) |
PF01773.17 | Nucleos_tra2_N | 110 | 18 | 92 | 0.44 (0.28-0.61) |
PF07662.10 | Nucleos_tra2_C | 110 | 18 | 92 | 0.44 (0.28-0.61) |
PF13807.3 | GNVR | 25 | 3 | 22 | 0.44 (0.16-0.71) |
PF01062.18 | Bestrophin | 10 | 2 | 8 | 0.44 (0.00-0.90) |
PF02163.19 | Peptidase_M50 | 43 | 4 | 39 | 0.44 (0.12-0.75) |
PF02706.12 | Wzz | 34 | 4 | 30 | 0.43 (0.20-0.67) |
PF01027.17 | Bax1-I | 68 | 16 | 52 | 0.42 (0.26-0.58) |
PF04235.9 | DUF418 | 7 | 1 | 6 | 0.42 (0.42-0.42) |
PF02397.13 | Bac_transf | 11 | 1 | 10 | 0.40 (0.40-0.40) |
PF00953.18 | Glycos_transf_4 | 86 | 3 | 83 | 0.39 (0.18-0.61) |
PF01790.15 | LGT | 75 | 12 | 63 | 0.39 (0.21-0.58) |
PF01252.15 | Peptidase_A8 | 63 | 22 | 41 | 0.39 (0.23-0.55) |
PF10604.6 | Polyketide_cyc2 | 12 | 5 | 7 | 0.39 (0.11-0.66) |
PF07264.8 | EI24 | 73 | 38 | 35 | 0.36 (0.23-0.50) |
PF04138.11 | GtrA | 18 | 2 | 16 | 0.36 (0.00-0.79) |
PF17154.1 | GAPES3 | 8 | 1 | 7 | 0.36 (0.36-0.36) |
PF07298.8 | NnrU | 43 | 5 | 38 | 0.35 (0.15-0.56) |
PF16401.2 | DUF5009 | 12 | 3 | 9 | 0.35 (0.04-0.66) |
PF03609.11 | EII-Sor | 22 | 12 | 10 | 0.33 (0.10-0.57) |
PF12811.4 | BaxI_1 | 20 | 2 | 18 | 0.33 (0.02-0.65) |
PF04116.10 | FA_hydroxylase | 85 | 7 | 78 | 0.31 (0.04-0.59) |
PF02417.12 | Chromate_transp | 8 | 1 | 7 | 0.29 (0.29-0.29) |
PF01059.14 | Oxidored_q5_N | 27 | 2 | 25 | 0.20 (0.00-0.50) |
PF06942.9 | GlpM | 6 | 5 | 1 | 0.20 (0.20-0.20) |
PF07331.8 | TctB | 6 | 1 | 5 | 0.20 (0.20-0.20) |
PF06800.9 | Sugar_transport | 29 | 2 | 27 | 0.19 (0.05-0.33) |
PF02654.12 | CobS | 6 | 1 | 5 | 0.00 (0.00-0.00) |
PF06966.9 | DUF1295 | 10 | 1 | 9 | 0.00 (0.00-0.00) |
1 A statistical model for improved membrane protein expression using sequence-derived features
Adapted from Saladi SM, Javed N, Müller A, Clemons WM. 2018. A statistical model for improved membrane protein expression using sequence-derived features. J Biol Chem 293:4913–4927. doi:10.1074/jbc.RA117.001052
1.1 Abstract
The heterologous expression of integral membrane proteins (IMPs) remains a major bottleneck in the characterization of this important protein class. IMP expression levels are currently unpredictable, which renders the pursuit of IMPs for structural and biophysical characterization challenging and inefficient. Experimental evidence demonstrates that changes within the nucleotide or amino-acid sequence for a given IMP can dramatically affect expression levels; yet these observations have not resulted in generalizable approaches to improve expression levels. Here, we develop a data-driven statistical predictor named IMProve, that, using only sequence information, increases the likelihood of selecting an IMP that expresses in E. coli. The IMProve model, trained on experimental data, combines a set of sequence-derived features resulting in an IMProve score, where higher values have a higher probability of success. The model is rigorously validated against a variety of independent datasets that contain a wide range of experimental outcomes from various IMP expression trials. The results demonstrate that use of the model can more than double the number of successfully expressed targets at any experimental scale. IMProve can immediately be used to identify favorable targets for characterization. Most notably, IMProve demonstrates for the first time that IMP expression levels can be predicted directly from sequence.
1.2 Introduction
The biological importance of integral membrane proteins (IMPs) motivates structural and biophysical studies that require large amounts of purified protein at considerable cost. Only a small percentage can be produced at high-levels resulting in IMP structural characterization lagging far behind that of soluble proteins; IMPs currently constitute less than 2% of deposited atomic-level structures (Hendrickson, 2016). To increase the pace of structure determination, the scientific community created large government-funded structural genomics consortia facilities, like the NIH-funded New York Consortium on Membrane Protein Structure (NYCOMPS) (Punta et al., 2009). For this representative example, more than 8000 genes, chosen based on characteristics hypothetically related to success, yielded only 600 (7.1%) highly expressing proteins (Love et al., 2010) resulting to date in 34 (5.6% of expressed proteins) unique structures (based on annotation in the RCSB PDB (Berman et al., 2000)). This example highlights the funnel problem of structural biology, where each stage of the structure pipeline eliminates a large percentage of targets compounding into an overall low rate of success (Lewinson et al., 2008). With new and rapidly advancing technologies like cryo-electron microscopy, serial femtosecond crystallography, and micro-electron diffraction, we expect that the latter half of the funnel, structure determination, will increase in success rate (Johansson et al., 2017; Merk et al., 2016; Nannenga and Gonen, 2016). However, IMP expression levels will continue to limit targets accessible for study (Bill et al., 2011).
Tools are needed for improving the number of IMPs with successful heterologous expression (we use expression synonymously for the term ‘overexpression’ in the field). While significant work has shown promise on a case-by-case basis, e.g., growth at lower temperatures, codon optimization (Nørholm et al., 2012), 5’ UTR randomization (Mirzadeh et al., 2015), and regulating transcription (Wagner et al., 2008), a generalizable solution remains elusive. Several RNA structure prediction methods have been used to explain or predict soluble protein expression levels (Price et al., 2009; Reis and Salis, 2020). For individual IMPs, simple changes, can have dramatic effects on the amount of expressed proteins—only partially explained by computational methods in the case of modifications at the 5’ region (Mirzadeh et al., 2015; Sarkar et al., 2008; Schlinkmann et al., 2012). Broadly, each new IMP target must be addressed individually as the conditions that were successful for a previous target seldom carry over to other proteins, even amongst closely related homologs (Lewinson et al., 2008; Marshall et al., 2016).
Considering the scientific value of IMP studies, it is surprising that there are no methods that can provide solutions for improved expression outcomes with broad applicability across protein families and genomes. There are no approaches that can decode sequence-level information for predicting IMP expression; yet it is common knowledge that sequence changes which alter overall biophysical features of the protein and mRNA transcript can measurably influence IMP biogenesis. While physics-based approaches which have proven successful in correlating integration efficiency and expression (Marshall et al., 2016; Niesen et al., 2017), that and other work revealed that simple application of specific ‘sequence features’ are inadequate to predict IMP expression (Daley et al., 2005; Nørholm et al., 2013). As an example, while the positive-inside rule is an important indicator of proper IMP biogenesis, the number of positive-charges on cytoplasmic loops alone does not predict expression level (Seppälä et al., 2010; Van Lehn et al., 2015). The reasons for this failure to connect sequence to expression likely lie in the complex underpinnings of IMP biogenesis, where the interplay between many sequence features at both the protein and nucleotide levels must be considered. Optimizing for a single sequence feature likely diminishes the beneficial effect of other features (e.g., increasing TM hydrophobicity might diminish favorable mRNA properties). Without accounting for the broad set of sequence features related to IMP expression, it is impossible to predict differences in expression levels.
Development of a low-cost, computational resource that significantly and reliably predicts improved expression outcomes would transform the study of IMPs. Attempts to develop such algorithms have so far failed. Several examples, Daley, von Heijne, and coworkers (Daley et al., 2005; Nørholm et al., 2013) as well as NYCOMPS, were unable to use experimental expression data sets to train models that returned any predictive performance (personal communication). This is not surprising, given the difficulty of expressing IMPs and the limits in the knowledge of the sequence features that drive expression. In other contexts, statistical tools based on sequence have been shown to work; for example, those developed to predict soluble protein expression and/or crystallization propensities (Bertone et al., 2001; Jahandideh et al., 2014; Price et al., 2009). Such predictors are primarily based on available experimental results from the Protein Structure Initiative (Chen et al., 2004; Gabanyi et al., 2011). While collectively these methods have supported significant advances in biochemistry, none of the models are able to predict IMP outcomes due to limitations inherent in the model development process. As IMPs have an extremely low success rate, they are either explicitly excluded from the training process or are implicitly down-weighted by the statistical model (for representative methodology see (Slabinski et al., 2007a)). Consequently, none have successfully been able to map IMP expression to sequence.
Here, we demonstrate for the first time that it is possible to predict IMP expression directly from sequence. The resulting predictor allows one to enrich expression trials for proteins with a higher probability of success. To connect sequence to prediction, we develop a statistical model that maps a set of sequences to experimental expression levels via calculated features—thereby simultaneously accounting for the many potential determinants of expression. The resulting IMProve model allows ranking of any arbitrary set of IMP sequences in order of their relative likelihood of successful expression. The IMProve model is extensively validated against a variety of independent datasets demonstrating that it can be used broadly to predict the likelihood of expression in E. coli of any IMP. With IMProve, we have built a way for more than two-fold enrichment of positive expression outcomes relative to the rate attained from the current method of randomly selecting targets. We highlight how the model informs on the biological underpinnings that drive likely expression. Finally, we provide direct examples where the model can be used for a typical researcher. Our novel approach and the resulting IMProve model provide an exciting paradigm for connecting sequence space to complex experimental outcomes.
1.3 Results
For this study, we focus on heterologous expression in E. coli, due to its ubiquitous use as a host across the spectrum of the membrane proteome. Low cost and low barriers for adoption highlight the utility of E. coli as a broad tool if the expression problem can be overcome. Furthermore, proof-of-principle in E. coli will illustrate methodology for constructing similar predictive methods for expressing IMPs in other hosts (e.g., yeast, insect cells).
1.3.1 Development of a computational model trained on E. coli expression data
A key component of any data-driven statistical model is the choice of dataset used for training. Having searched the literature, we identified two publications that contained quantitative datasets on the IPTG-induced overexpression of E. coli polytopic IMPs in E. coli. The first set, Daley, Rapp et al., contained activity measures, proxies for expression level, from C-terminal tags of either GFP or PhoA (alkaline phosphatase)(Daley et al., 2005). The second set, Fluman et al., used a subset of constructs from the first and contained a more detailed analysis utilizing in-gel fluorescence to measure folded protein (Fluman et al., 2014) (see Section 1.5.4). The expression levels strongly correlated (Spearman’s \(\rho\) = 0.73) between the two datasets demonstrating that normalized GFP activity was a good measure of the amount of folded IMP (Figure 1.1A and (Fluman et al., 2014; Geertsma et al., 2008)). The experimental set-up employed multiple 96-well plates over multiple days resulting in pronounced variability in the absolute expression level of a given protein between trials. Daley, Rapp et al. calculated average expression levels by dividing the raw expression level of each protein by that of a control protein on the corresponding plate.
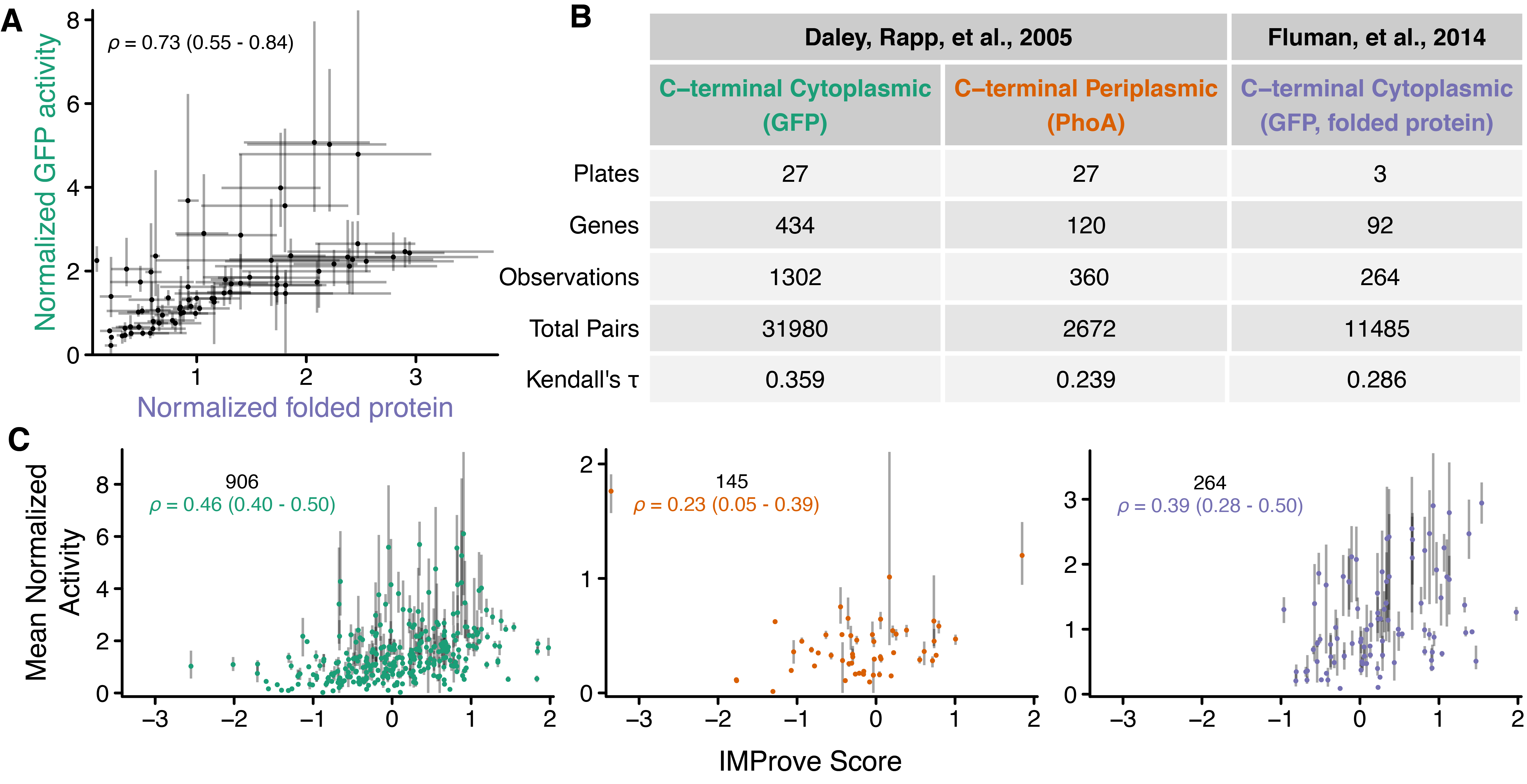
To successfully map sequence to expression, we additionally needed to derive numerical features from a given gene sequence that have been empirically related to expression. 87 sequence features from protein and nucleotide sequence were calculated for each gene using custom code together with published software (codonW (Peden, 2000), tAI (dos Reis et al., 2003), NUPACK (Zadeh et al., 2011), Vienna RNA (Lorenz et al., 2011), Codon Pair Bias (Coleman et al., 2008), Disembl (Linding et al., 2003), and RONN (Yang et al., 2005)). Relative metrics (e.g., codon adaptation index) are calculated with respect to the E. coli K-12 substr. MG1655 (Zhou and Rudd, 2013) quantity. The octanol-water partitioning (Wimley et al., 1996), GES hydrophobicity (Engelman et al., 1986), \(\Delta G\) of insertion (Hessa et al., 2007) scales were employed as well. Transmembrane segment topology was predicted using Phobius constrained for the training data and Phobius for all other datasets (Käll et al., 2004). Two RNA secondary structure metrics were prompted in part by Goodman, et al. (Goodman et al., 2013). Table 1.1 includes a detailed description of each feature. All features are calculated solely from the coding region of each gene of interest excluding other portions of the open reading frame and plasmid (e.g., linkers and tags, 5’ untranslated region, copy number).
Fitting the data to a simple linear regression provides a facile method for deriving a weight for each feature. However, using the set of sequence features, we were unable to successfully fit a linear regression using the normalized GFP and PhoA measurements reported in the Daley, Rapp et al. study. Similarly, using the same feature set and data, we were unable to train a standard linear Support Vector Machine (SVM) to predict the expression data either averaged or across all plates (see Table 1.1; Section 1.5.2, Section 1.5.3). Due to the attempts by others to fit this data, this outcome may not be surprising and suggested that a more complex analysis was required.
We hypothesized that training on relative measurements across the entire dataset introduced errors that were limiting. To address this, we instead only compare measurements within an individual plate, where differences between trials are less likely to introduce errors. To account for this, a preference-ranking linear SVM algorithm (SVMrank (Tsochantaridis et al., 2005)) was chosen (see Section 1.5.4). Simply put, the SVMrank algorithm determines the optimal weight for each sequence feature to best rank the order of expression outcomes within each plate over all plates, which results in a model where higher expressing proteins have higher scores. The outcome is identical in structure to a multiple linear regression, but instead of minimizing the sum of squared residuals, the SVMrank cost function accounts for the plate-wise constraint specified above. In practice, the process optimizes the correlation coefficient Kendall’s \(\tau\) (Equation 1.1) to converge upon a set of weights.
\[ \tau_{\text{kendall}} = \frac{\text{N}_\text{correctly ordered pairs} - \text{N}_\text{swapped pairs}}{\text{N}_\text{total pairs}} \tag{1.1}\]
The SVMrank training metric shows varying agreement for all three groups (i.e., \(\tau_{\text{kendall}} > 0\)) (Figure 1.1B). For individual genes, activity values normalized and averaged across trials were not directly used for the training procedure (see Section 1.5.4); yet one would anticipate that scores for each gene should broadly correlate with the expression average. Indeed, the observed normalized activities positively correlate with the score (dubbed IMProve score for Integral Membrane Protein expression improvement) output by the model (Figure 1.1C, \(\rho > 0\)). Since SVMrank transforms raw expression levels within each plate to ranks before training, Spearman’s \(\rho\), a rank correlation coefficient describing the agreement between two ranked quantities, is better suited for quantifying correlation over more common metrics like the \(R^2\) of a regression and Pearson’s \(r\). While these metrics demonstrate that the model can rank expression outcomes across all proteins in the training set, for PhoA-tagged proteins, the model appears less successful. The implications for this are discussed later (see Figure 1.2G).
1.3.2 Demonstration of prediction against an independent large expression dataset
While the above analyses show that the model successfully fits the training data, we assess the broader applicability of the model outside the training set based on its success at predicting the outcomes of independent expression trials from distinct groups and across varying scales. The first test considers results from NYCOMPS, where 8444 IMP genes entered expression trials, in up to eight conditions, resulting in 17114 expression outcomes (Figure 1.2A) (Punta et al., 2009). The majority of genes were attempted in only one condition (Figure 1.2B), and, importantly, outcomes were non-quantitative (binary: expressed or not expressed) as indicated by the presence of a band by Coomassie staining of an SDS-PAGE gel after small-scale expression, solubilization, and nickel affinity purification (Love et al., 2010). For this analysis, the experimental results are either summarized as outcomes per gene or broken down as raw outcomes across defined expression conditions. For outcomes per gene, we can consider various thresholds for considering a gene as positive based on NYCOMPS expression success (Figure 1.2B). The most stringent threshold only regards a gene as positive if it has no negative outcomes (“Only Positive”, Figure 1.2B, red). Since a well expressing gene would generally advance in the NYCOMPS pipeline without further small-scale expression trials, this positive group likely contains the best expressing proteins. A second category comprises genes with at least one positive and at least one negative trial (“Mixed”, Figure 1.2B, blue). These genes likely include proteins that are more difficult to express.
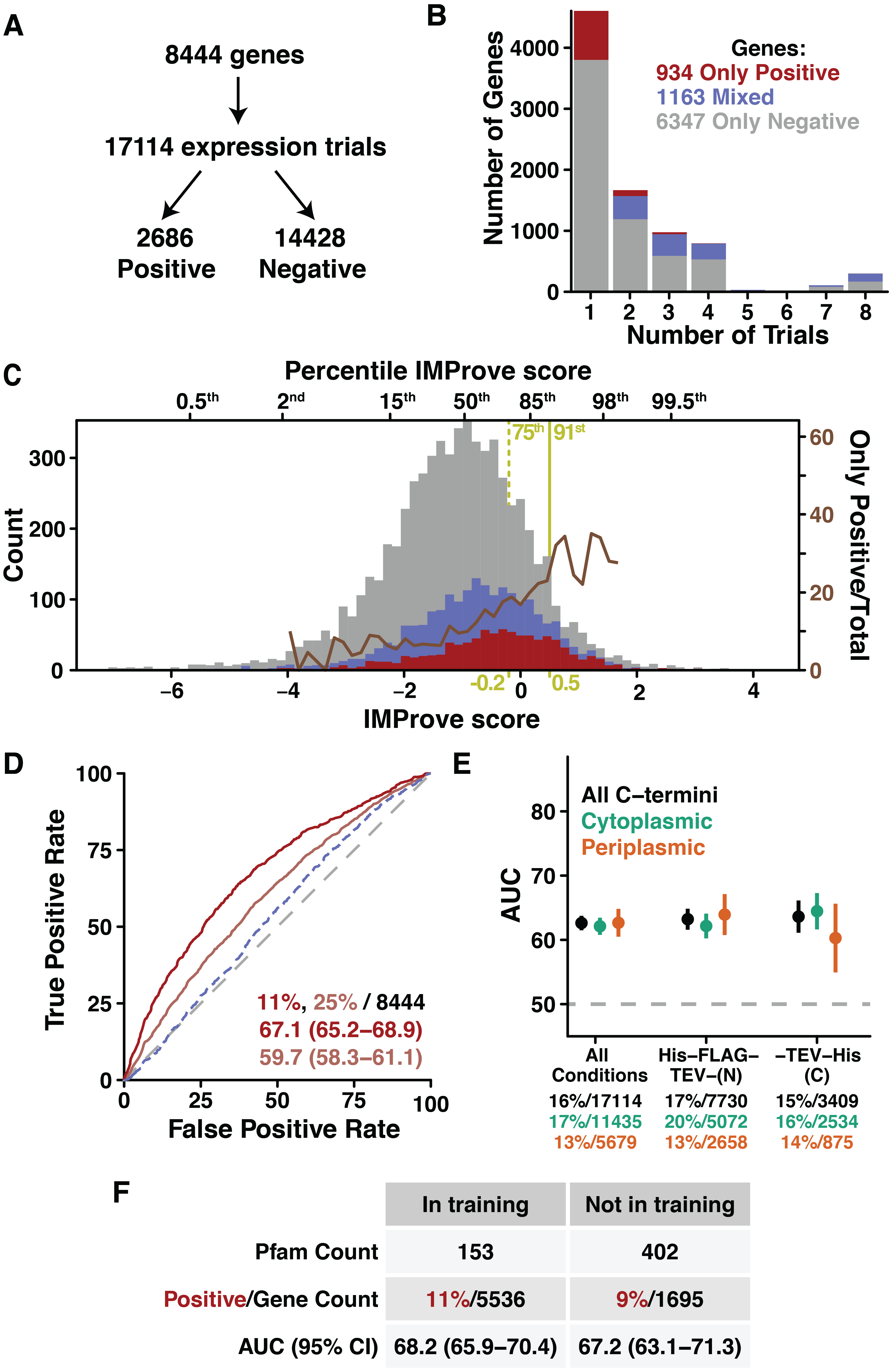
A histogram of the IMProve scores for genes separated by expression success shows predictive power of the IMProve model (Only Positive, red; Mixed, blue; Only Negative, grey) (Figure 1.2C). Visually, the distribution of the scores for the Only Positive group is shifted to a higher score relative to the Only Negative and Mixed groups. The dramatic increase in the percentage of Only Positive genes as a function of increasing IMProve score (overlaid as a brown line) further emphasizes this. In fact, simply selecting the top 25% of proteins by IMProve score (-0.2 or higher, Figure 1.2C, yellow dotted line) would have increased the number of positive outcomes from 11% to 20%, a 1.8 fold improvement.
While this is suggestive, we aim to more systematically assess predictive power. For NYCOMPS and subsequent data sets, the outcomes are either binary (e.g., in NYCOMPS expressed or not expressed) or categorical (e.g., arbitrary ranked scale), correlation coefficients cannot be used here. Instead, we turn to an alternative framework: the Receiver Operating Characteristic (ROC). ROC curves quantify the tradeoff between true positive and false positive predictions across numerical scores from a predictor. The figure of merit is the Area Under the ROC Curve (AUC), where a perfect predictor has an AUC = 100% and a random predictor has an AUC = 50 % (Figure 1.2D, grey dashed line). An AUC greater than 50% indicates that a model is predictive (percentage signs hereafter omitted) (see Section 1.5.5 and (Swets et al., 2000)). We will use the ROC framework to quantitatively validate the ability of our model to predict protein expression data—all independent of the training process.
Returning to the NYCOMPS data, ROC analysis shows that the IMProve model markedly distinguishes genes in the most stringent positive group (Only Positive) from all other genes (AUC = 67.1) (Figure 1.2C red). A permissive threshold considering genes as positive with at least one positive trial (Only Positive plus Mixed genes) shows moderate predictive power (Figure 1.2C pink, AUC = 59.7). If instead the Mixed genes are considered alone (excluding the Only Positive), the model very weakly distinguishes the Mixed group from the Only Negative genes (Figure 1.2C dashed blue, AUC = 53.5). This likely supports the notion that the Mixed pool largely consists of more difficult-to-express genes.
We next confirm the ability of the IMProve model to predict within plasmids or sequence space distinct from those within the limited training set. For an overfit model, one might expect that only the subset of targets which most closely mirror the training data would show strong prediction. On the contrary, the model shows consistent performance throughout each of the eight distinct experimental conditions tested (Figure 1.2G and Table 1.2). One may also consider that the small size of the training set limited the number of protein folds sampled and, therefore, limited the number of folds that could be predicted by the model. To test this, we consider the performance of the model with regards to protein homology families, as defined by Pfam family classifications (Finn et al., 2014). The 8444 genes in the NYCOMPS dataset fall into 555 Pfam families (~15% not classified). To understand whether the IMProve score is biased towards families present in the training set, we separate genes in the NYCOMPS dataset into either within the 153 Pfam families found in the training set or outside this pool (i.e., not in the training set). Satisfyingly, there is no significant difference in AUC at 95% confidence between these groups (68.2 versus 67.2) (Figure 1.2H). Combined, this highlights that the model is not sensitive to the experimental design of the training set and predicts broadly across different vector backbones and protein folds.
The ability to predict the experimental data from NYCOMPS allows returning to the question of alkaline phosphatase as a metric for expression. For the training set, proteins with C-termini in the periplasm show weaker fitting by the model (Figure 1.1, orange). To assess the generality of this result, the NYCOMPS outcomes are split into pools for either cytoplasmic or periplasmic C-terminal localization and AUCs are calculated for each. There are no significant differences in predictive capacity across all conditions (Figure 1.2G, green vs. orange) irrespective of whether the tag is at the N- or C-terminus. This demonstrates that the IMProve model is applicable for all topologies.
At this point, it is useful to consider the potential improvement in the number of positive outcomes by using the IMProve model. NYCOMPS tested about a tenth of the 74 thousand possible IMPs from the 98 genomes of interest for expression (Punta et al., 2009). Had NYCOMPS tested the same number of genes from this pool, but selected to have an IMProve score greater than 0.5 (at the 91st percentile (Figure 1.2C, yellow solid line)), they would have increased their positive pool of 934 by an additional 1207 proteins. This represents a more than two-fold improvement in the return on investment and is a clear benchmark of success for the IMProve model.
1.3.3 Further demonstration of prediction against small-scale independent datasets
The NYCOMPS example demonstrates the predictive power of the model across the broad range of sequence space encompassed by that dataset. Next, the performance of the model is tested against relevant subsets of sequence space (e.g., a family of proteins or the proteome from a single organism), which are reminiscent of laboratory-scale experiments that precede structural or biochemical analyses. While a number of datasets exist (Bernaudat et al., 2011; Eshaghi et al., 2005; Gordon et al., 2008; Korepanova et al., 2005; Lewinson et al., 2008; Lundstrom et al., 2006; Ma et al., 2013; Madhavan et al., 2010; Petrovskaya et al., 2010; Surade et al., 2006; Szakonyi et al., 2007), we identified seven for which complete sequence information could be obtained to calculate all the necessary sequence features (Korepanova et al., 2005; Lundstrom et al., 2006; Ma et al., 2013; Madhavan et al., 2010; Surade et al., 2006).
To understand the predictive performance within each of the small-scale datasets, we analyze the predictive performance of the model and highlight how the model could have been used to streamline those experiments. The clear predictive performance within the large-scale NYCOMPS dataset (Figure 1.2) serves as a benchmark of expected performance at the scale of the experimental efforts for an individual lab (Figure 1.3A). As targets within the various datasets were tested only one or a few times, experimental variability within each set could play a large-role on the outcomes noted. Therefore, we summarize positives within each dataset as those genes with the highest level of outcome as reported by the original authors as this outcome is likely most robust to such variability (e.g., seen via Coomassie Blue staining of an SDS-PAGE gel). To be complete, we have plotted and considered predictive performance across all possible outcomes as well (Figure 1.3B-D, Figure 1.6, Figure 1.7).
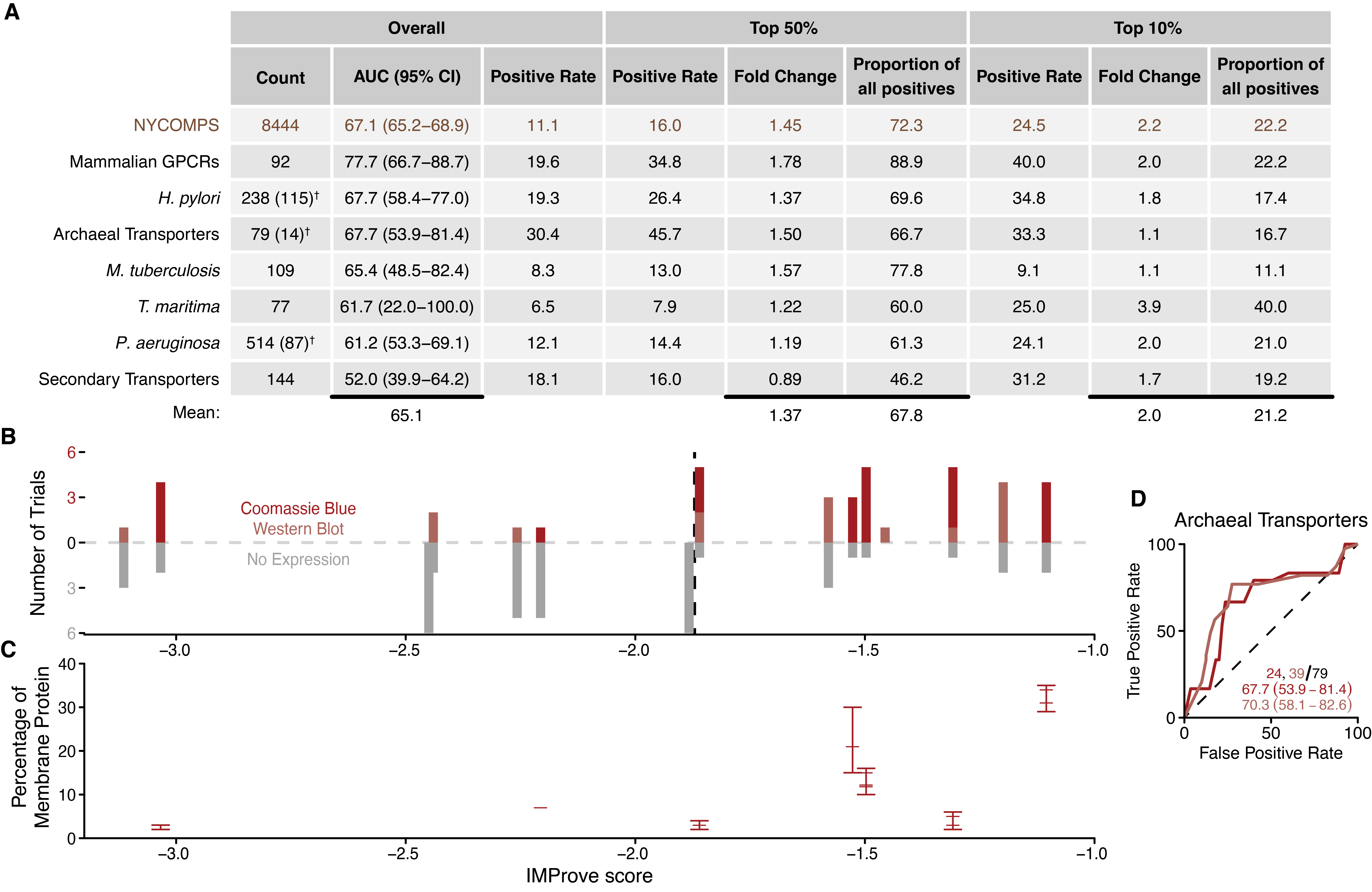
The performance of the IMProve model for each of the small-scale datasets is consistent with that seen for the NYCOMPS dataset (Figure 1.3A). This is most directly indicated by a mean AUC across all datasets of 65.6, highlighting the success across the varying scales. While the overall positive rate is different for each dataset, considering a cut-off in IMProve score, e.g., the top 50% or 10% of targets ranked by score, would have resulted in a greater percentage of positive outcomes. On average, ~70% of positives are captured within the top half of scores. Similarly, for the top 10% of scores, on average over 20% of the positives are captured. Simply put, for one tenth of the work one would capture a significant number of the positive outcomes within the pool of targets in each dataset.
For broader consideration, one can consider the fold change in positive rate by selecting targets informed by IMProve scores. Using the data available, only testing proteins within the top 10% of scores would result in an average fold change of 2.0 in the positive rate (i.e., twice as many positively expressed proteins). As positive rate is a bounded quantity (maximum is 100%), the possible fold change is bounded as well and becomes relative to the overall positive rate when considering various cut-offs (e.g., for T. maritima the maximum fold-change is 15.4 while for archaeal transporters it is 3.3). Taking the average maximum possible fold change (7.5), the IMProve model achieves nearly a third of the possible improvement in positive rate compared to a perfect predictor.
Since the IMProve model was trained on quantitative expression levels, we also expect that it captures quantitative trends in expression, i.e., a higher score translates to greater amount of expressed protein. While the NYCOMPS results are consistent with this (Figure 1.2b), of the various data sets, only the expression of archaeal transporters presents quantitative outcomes for consideration. For this dataset, 14 archaeal transporters were chosen based on their homology to human proteins (Ma et al., 2013) and tested for expression in E. coli; total protein was quantified in the membrane fraction by Coomassie Blue staining of an SDS-PAGE gel. Here, the majority of the expressing proteins fall into the higher half of the IMProve scores, 7 out of 9 of those with multiple positive outcomes (Figure 1.3B). Strikingly, quantification of the Coomassie Blue staining highlights a clear correlation with the IMProve score where the higher expressing proteins have higher scores (Figure 1.3C).
A final test considers the ability of the model to predict expression in hosts other than E. coli. The expression trials of 101 mammalian GPCRs in bacterial and eukaryotic systems (Lundstrom et al., 2006) provides a data set for considering this question. For this experiment, trials in E. coli clearly follow the trend that IMProve can predict within this group of mammalian proteins (AUC = 77.7) (Figure 1.3A, Figure 1.6A,B). However, the expression of the same set of proteins in P. pastoris fails to show any predictive performance (AUC = 54.8) (Figure 1.6A,B). This lack of predictive performance in P. pastoris suggests that the parameterization of the model, calibrated for E. coli expression, requires retraining to generate a different model that captures the distinct interplay of sequence parameters in other hosts.
1.3.4 Biological importance of various sequence features
Considering the success of IMProve, one might anticipate that biological properties driving prediction may provide insight into IMP biogenesis and expression. To derive principles about which features drive prediction, most statistical models (including SVMrank as used here) require that each data point and its features are drawn identically and distributed independently from an underlying distribution (referred to as “i.i.d.”). In the case of a linear model, if the features meet the i.i.d. criteria then extracting the importance of each feature is straightforward; the weight assigned to each feature corresponds to its relative importance. However, in our case, the input features do not meet the i.i.d. criteria because significant correlation exists between individual features (Figure 1.8).
Since overlapping sets of correlating features represent biological properties, the importance of a given biological property is distributed across many features. The result is that one cannot directly determine the biological underpinnings of the IMProve score. For example, the importance of transmembrane segment hydrophobicity for membrane partitioning is likely distributed between several features: among these the average \(\Delta \text{G}_{\text{insertion}}\) (Hessa et al., 2007) of TM segments has a positive weight whereas average hydrophobicity, a correlating feature, has a negative weight (Table 1.1, Figure 1.8). While this is counterintuitive, it is consistent with the expectation that the overall importance of transmembrane segment hydrophobicity is the sum of many correlated features. While one cannot disentangle the correlation between features, we attempt to address this complication by coarsening our view of the features to two levels: First, we analyze features derived from protein versus those derived from nucleotide sequence, and then we look more closely at features groups after categorizing by biological property.
The coarsest view of the features is a comparison of those derived from protein sequence versus those derived from nucleotide sequence. The summed weight for protein features is around zero, whereas for nucleotide features the summed weight is slightly positive suggesting that in comparison these features may be more important to the predictive performance of the model (Figure 1.4A). Within the training set, protein features more completely explain the score both via correlation coefficients (Figure 1.4B). However, comparison of the predictive performance of the two subsets of weights shows that the nucleotide features alone can give similar performance to the full model for the NYCOMPS dataset (Figure 1.4C). Within the small-scale datasets investigated, using only protein or nucleotide features shows no significant difference in predictive power at 95% confidence (Figure 1.4D). In general, this suggests that neither protein nor nucleotide features are solely responsible for successful IMP expression. However, within the context of the trained model, nucleotide features are critical for predictive performance for a large and diverse dataset such as NYCOMPS. This finding corroborates growing literature that the nucleotide sequence holds significant determinants of biological processes (Chartron et al., 2016; Fluman et al., 2014; Gamble et al., 2016; Goodman et al., 2013; Li et al., 2012).
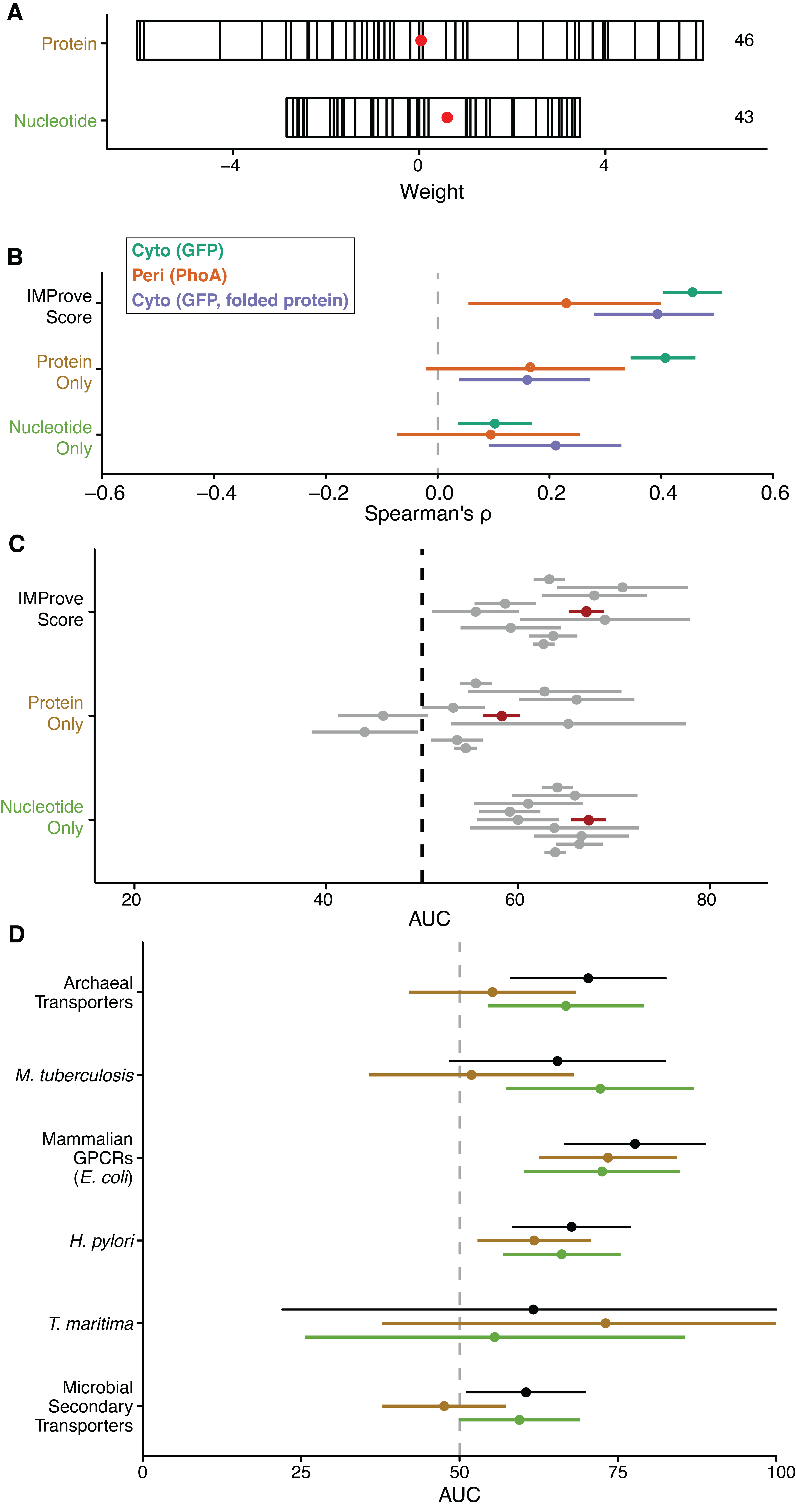
We next collapse conceptually similar features into biological categories that allow us to infer the phenomena that drive prediction. Categories are chosen empirically (e.g., the hydrophobicity group incorporates sequence features such as average hydrophobicity, maximum hydrophobicity, \(\Delta \text{G}_{\text{insertion}}\)), which results in a reduction in overall correlation (Figure 1.9A). The full category list is provided in Table 1.1. To visualize the importance of each category, the collapsed weights are summarized in Figure 1.9B, where each bar contains individual feature weights within a category. Features with a negative weight are stacked to the left of zero and those with a positive weight are stacked to the right. A red dot represents the sum of all weights, and the length of the bar gives the total absolute value of the combined weights within a category. Ranking the categories based on the sum of their weight suggests that some categories play a more prominent role than others. These include properties related to transmembrane segments (hydrophobicity and TM size/count), codon pair score, loop length, and overall length/pI.
To explore the role of each biological category in prediction, the performance of the model is assessed using only features within a given category. First, the strength of the correlation coefficients for given categories within the training set suggests the relative utility of each category for prediction. (Figure 1.9C, as in Figure 1.4B). Examples of categories with high correlation coefficients are 5’ Codon Usage, Length/pI, Loop Length, and SD-like Sites. To verify their importance for prediction, we consider the AUC for prediction using each feature category for the NYCOMPS dataset (Figure 1.9D). In this analysis, only Length/pI shows some predictive power. Overall, the analysis of the training and large-scale testing dataset shows that no feature category independently drives the predictor. Excluding each individually does not significantly affect the overall predictive performance, except for Length/pI (data not shown). Sequence length composes the majority of the weight within this category and is one of the highest weighted features in the model (Figure 1.9A). This is consistent with the anecdotal observation that larger IMPs are typically harder to express. However, this parameter alone would not be useful for predicting within a smaller subset, like a single protein family, where there is little variance in length (e.g., Figure 1.3,Figure 1.5). One might develop a predictor that was better for a given protein family under certain conditions with a subset of the entire features considered here; yet this would require a priori knowledge of the system, i.e., which sequence features were truly most important, and would preclude broad generalizability as shown for the IMProve model.
1.3.5 Usage of the IMProve model for IMP expression
We illustrate the IMProve model’s ability to identify promising homologs within a protein family by considering subsets of the broad range of targets tested by NYCOMPS. First, we consider two examples for protein families that do not have associated atomic resolution structures: copper resistance proteins (CopD, PF05425) and short-chain fatty-acid transporters (AtoE, PF02667). In the first two rows of Figure 1.5A, genes from the two families are plotted by IMProve score and colored by experimental outcome. In both cases, as indicated by the AUCs of 88.2 and 80.7 (Figure 1.5A), the model excels at predicting these families and provides a clear score cut-off to guide target selection for future expression experiments. For example, we expect that CopD homologs with IMProve scores above -1 will have a higher likelihood of expressing over other homologs.
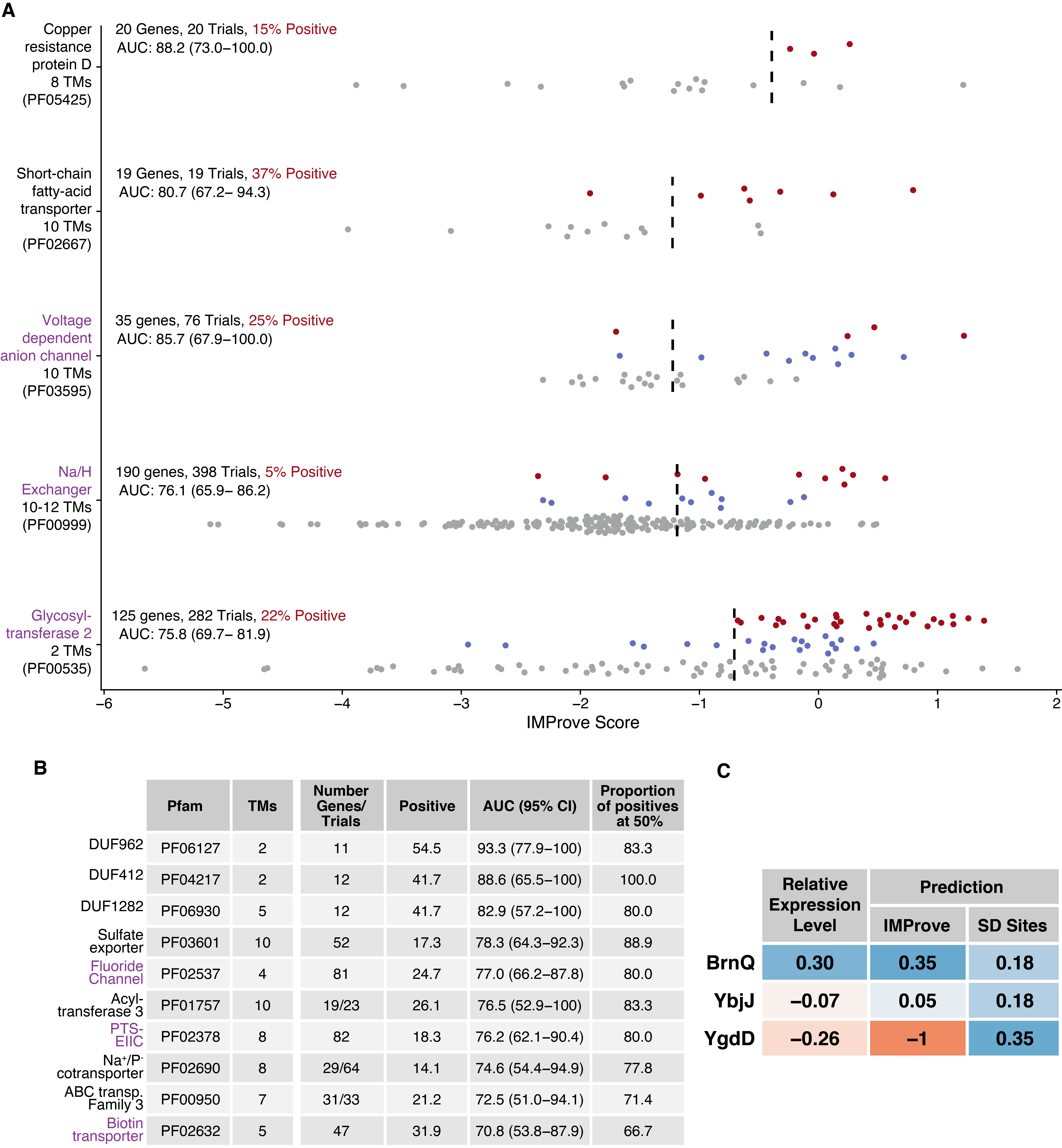
We have calculated predictive performance for each Pfam found in the NYCOMPS data which allows us to provide considerations for future experiments (Table 1.3). In particular, we highlight three families with many genes tested, multiple experimental trials and a spread of outcomes: voltage-dependent anion channels (PF03595), Na/H exchangers (PF00999), and glycosyltransferases (PF00535). For these, a very clear IMProve score cut-off emerges from the experimental outcomes (dashed line in Figure 1.5A). Strikingly, for these families the IMProve model clearly ranks the targets with Only Positive outcomes (red) at higher scores, again suggesting a preference for the best expressing proteins (see Figure 1.2 and Figure 1.3). Similarly, many more families within NYCOMPS are predicted with high statistical confidence (Table 1.3); we provide a subset as Figure 1.5B. For these, if only genes in the top 50% of IMProve score were tested, 81% of the total positives would be captured. As noted before, this is a dramatic increase in efficiency. Excitingly, many of these families remain to be resolved structurally. Considering these results with the broader experimental data sets (Figure 1.3), no matter the number of proteins one is willing to test, the IMProve model enables selecting targets with a high probability of expression success in E. coli.
1.3.6 Sequence optimization for expression
The predictive performance of the model implies that the features defined here provide a coarse approximation of the fitness landscape for IMP expression. Attempting to optimize a single feature by modifying the sequence will likely affect the resulting score and expression due to changes in other features. Fluman, et al. provides an illustrative experiment (Fluman et al., 2014). For that work, it was hypothesized that altering the number of Shine-Dalgarno (SD)-like sites in the coding sequence of a IMP would affect expression level. To test this, silent mutations were engineered within the first 200 bases of three proteins (genes ygdD, brnQ, and ybjJ from E. coli) to increase the number of SD-like sites with the goal of improving expression. Expression trials demonstrated that only one of the proteins (BrnQ) had improved expression of folded protein. While the number of SD-like sites alone does not correlate, only 1 out of 3, the resulting changes in the IMProve score correlate with the changes in measured expression level, 3 out of 3 (Figure 1.5C). The IMProve model’s ability to capture the outcomes in this small test case illustrates the utility of integrating the contribution of the numerous parameters involved in IMP biogenesis.
1.4 Discussion
Here, we have demonstrated a statistically driven predictor, IMProve, that decodes from sequence the sum of biological features that drive expression, a feat not previously possible (Nørholm et al., 2013). The current best practice for characterization of an IMP target begins with the identification and testing of multiple homologs or variants for expression. The predictive power of IMProve enables this by providing a low barrier-to-entry method to enrich for positive outcomes by more than two-fold. IMProve allows for the prioritization of targets to test for expression making more optimal use of limited human and material resources. For groups with small scale projects such as individual labs, this means that for the same cost one would double the success rate. For large scale groups, such as companies or consortia, IMProve can reduce by half the cost required to obtain the same number of positive results. We provide the current predictor as a web service where scores can be calculated, and the method, associated data, and suggested analyses are publically available to catalyze progress across the community (clemonslab.caltech.edu).
Having shown that IMP expression can be predicted, the generalizability of the model is remarkable despite several known limitations that will be investigated in next generation predictors. Using data from a single study for training precluded including certain features that empirically influence expression level such as fusion tags and the context of the protein in an expression plasmid, e.g., the 5’ untranslated region, for which there was no variation in the Daley, Rapp, et al. dataset. Moreover, using a simple proof-of-concept linear model allowed for a straightforward and robust predictor; however, intrinsically it cannot be directly related to the biological underpinnings. While we can extract some biological inference, a linear combination of sequence features does not explicitly reflect the reality of physical limits for host cells. To some extent, constraint information is likely encoded in the complex architecture of the underlying sequence space (e.g., through the genetic code, TM prediction, RNA secondary structure analyses). Future statistical models that improve on these limitations will hone predictive power and more intricately characterize the interplay of variables that underlie IMP expression. By building upon the methodology here and by careful analysis of datasets in the literature, we expect to train IMP expression predictors for other host systems (e.g., eukaryotic cells).
A surprising outcome of our results is the observation of a quantitatively important contribution of the nucleotide sequence as a component of the IMProve score. This echoes the growing literature that aspects of the nucleotide sequence are important determinants of protein biogenesis in general (Chartron et al., 2016; Fluman et al., 2014; Gamble et al., 2016; Goodman et al., 2013; Li et al., 2012). While one expects that there may be different weights for various nucleotide derived features between soluble and IMPs, it is likely that these features are important for soluble proteins as well. An example of this is the importance of codon optimization for soluble protein expression, which has failed to show any general benefit for IMPs (Nørholm et al., 2012). Current expression predictors that have predictive power for soluble proteins have only used protein sequence for deriving the underlying feature set (Slabinski et al., 2007b; Wang et al., 2016). Future prediction methods will likely benefit from including nucleotide sequence features as done here.
The ability to predict phenotypic results using sequence based statistical models opens a variety of opportunities. As done here, this requires a careful understanding of the system and its underlying biological processes enumerated in a multitude of individual variables that impact the stated goal of the predictor, in this case enriching protein expression. As new features related to expression are discovered, future work will incorporate these leading to improved models. This can include features derived from other approaches such as the integration efficiency from coarse-grained molecular dynamics (Marshall et al., 2016; Niesen et al., 2017) and 5’ mRNA secondary structure/translation initiation efficiency (Goodman et al., 2013; Mirzadeh et al., 2015; Reis and Salis, 2020). Based on these results, expanding to new expression hosts such as eukaryotes seems entirely feasible, although a number of new features may need to be considered, e.g., glycosylation sites and trafficking signals. Moreover, the ability to score proteins for expressibility creates new avenues to computationally engineer IMPs for expression. The proof-of-concept described here required significant work to compile data from genomics consortia and the literature in a readily useable form. As data becomes more easily accessible, broadly leveraging diverse experimental outcomes to decode sequence-level information, an extension of this work, is anticipated.
1.5 Materials and Methods
Sequence mapping & retrieval and feature calculation was performed in Python 2.7 (Van Rossum and Drake Jr, 1995) using BioPython (Cock et al., 2009) and NumPy (van der Walt et al., 2011); executed and consolidated using Bash (shell) scripts; and parallelized where possible using GNU Parallel (Tange, 2011). Data analysis and presentation was done in R (R Core Team, 2015) within RStudio (RStudio Team, 2015) using magrittr (Bache and Wickham, 2014), plyr (Wickham, 2011), dplyr (Wickham and Francois, 2015), asbio (Aho, 2015), and datamart (Weinert, 2014) for data handling; ggplot2 (Wickham, 2016, p. 2), ggbeeswarm (Clarke and Sherrill-Mix, 2015), GGally (Schloerke et al., 2016), gridExtra (Auguie, 2015), cowplot (Wilke, 2015), scales (Wickham, 2015), viridis (Garnier, 2016), and RColorBrewer (Harrower and Brewer, 2003; Neuwirth, 2014) for plotting; multidplyr (Wickham, n.d.) with parallel (R Core Team, 2015) and foreach (Revolution Analytics and Weston, 2015a) with iterators (Revolution Analytics and Weston, 2015b) and doMC (Revolution Analytics and Weston, 2015c)/doParallel (Revolution Analytics and Weston, 2015d) for parallel processing; and roxygen2 (Wickham et al., 2015) for code organization and documentation as well as other packages as referenced.
1.5.1 Collection of data necessary for learning and evaluation
E. coli Sequence Data
The nucleotide sequences from (Daley et al., 2005) were deduced by reconstructing forward and reverse primers (i.e., ~20 nucleotide stretches) from each gene in Colibri (based on EcoGene 11), the original source cited and later verified these primers against an archival spreadsheet provided directly by Daniel Daley (personal communication). To account for sequence and annotation corrections made to the genome after Daley, Rapp, et al.’s work, these primers were directly used to reconstruct the amplified product from the most recent release of the E. coli K-12 substr. MG1655 genome (Zhou and Rudd, 2013) (EcoGene 3.0; U00096.3). Although Daniel Daley mentioned that raw reads from the Sanger sequencing runs may be available within his own archives, it was decided that the additional labor to retrieve this data and parse these reads would not significantly impact the model. The deduced nucleotide sequences were verified against the protein lengths given in Supplementary Table 1 from (Daley et al., 2005). The plasmid library tested in (Fluman et al., 2014) was provided by Daniel Daley, and those sequences are taken to be the same.
E. coli Training Data
The preliminary results using the mean-normalized activities echoed the findings of (Daley et al., 2005) that these do not correlate with sequence features either in the univariate sense (many simple linear regressions, Supplementary Table 1 (Daley et al., 2005)) or a multivariate sense (multiple linear regression, data not shown). This is presumably due to the loss of information regarding variability in expression level for given genes or due to the increase in variance of the normalized quantity (See Section 1.5.4) due to the normalization and averaging procedure. Daniel Daley and Mikaela Rapp provided spreadsheets of the outcomes from the 96-well plates used for their expression trials and sent scanned copies of the readouts from archival laboratory notebooks where the digital data was no longer accessible (personal communication). Those proteins without a reliable C-terminal localization (as given in the original work) or without raw expression outcomes were not included in further analyses.
Similarly, Nir Fluman also provided spreadsheets of the raw data from the set of three expression trials performed in (Fluman et al., 2014).
New York Consortium on Membrane Protein Structure (NYCOMPS) Data
Brian Kloss, Marco Punta, and Edda Kloppman provided a dataset of actions performed by the NYCOMPS center including expression outcomes in various conditions (Love et al., 2010; Punta et al., 2009). The protein sequences were mapped to NCBI GenInfo Identifier (GI) numbers either via the Entrez system (Schuler et al., 1996) or the Uniprot mapping service (UniProt Consortium, 2012). Each GI number was mapped to its nucleotide sequence via a combination of the NCBI Elink mapping service and the “coded_by” or “locus” tags of Coding Sequence (CDS) features within GenBank entries. Though custom-purpose code was written, a script from Peter Cock is available to do the same task via a similar mapping mechanism (Cock, 2009). To confirm all sequences, the TargetTrack (Chen et al., 2004) XML file was parsed for the internal NYCOMPS identifiers and compared for sequence identity to those that had been mapped using the custom script; 20 (less than 1%) of the sequences had minor inconsistencies and were manually replaced.
Archaeal transporters Data
The locus tags (“Gene Name” in Table 1) were mapped directly to the sequences and retrieved from NCBI (Ma et al., 2013). Pikyee Ma and Margarida Archer clarified questions regarding their work to inform the analysis.
GPCR Expression Data
Nucleotide sequences were collected by mapping the protein identifiers given in Table 1 from (Lundstrom et al., 2006) to protein GIs via the Uniprot mapping service (UniProt Consortium, 2012) and subsequently to their nucleotide sequences via the custom mapping script described above (see NYCOMPS 1.5.1.3). The sequence length and pI were validated against those provided. Renaud Wagner assisted in providing the nucleotide sequences for genes whose listed identifiers were unable to be mapped and/or did not pass the validation criteria as the MeProtDB (the sponsor of the GPCR project) does not provide a public archive.
Helicobacter pylori Data
Nucleotide sequences were retrieved by mapping the locus tags given in Supplemental Table 1 from (Psakis et al., 2007) to locus tags in the Jan 31, 2014 release of the H. pylori 26695 genome (AE000511.1). To verify sequence accuracy, sequences whose molecular weight matched that given by the authors were accepted. Those that did not match, in addition to the one locus tag that could not be mapped to the Jan 31, 2014 genome version, were retrieved from the Apr 9, 2015 release of the genome (NC_000915.1). Both releases are derived from the original sequencing project (Tomb et al., 1997). After this curation, all mapped sequences matched the reported molecular weight.
In this data set, expression tests were performed in three expression vectors and scored as 1, 2, or 3. Two vectors were scored via two methods. For these two vectors, the two scores were averaged to give a single number for the condition making them comparable to the third vector while yielding 2 additional thresholds (1.5 and 2.5) result in the 5 total curves shown (Figure 1.8B).
Mycobacterium tuberculosis Data
The authors note using TubercuList through GenoList (Lechat et al., 2008), therefore, nucleotide sequences were retrieved from the archival website based on the original sequencing project (Cole et al., 1998). The sequences corresponding to the identifiers and outcomes in Table 1 from (Korepanova et al., 2005) were validated against the provided molecular weight .
Secondary Transporter Data
GI Numbers given in Table 1 from (Surade et al., 2006) were matched to their CDS entries using the custom mapping script described above (see NYCOMPS 1.5.1.3). Only expression in E. coli with IPTG-inducible vectors was considered.
Thermotoga maratima Data
Gene names given in Table 1 (Dobrovetsky et al., 2005) were matched to CDS entries in the Jan 31, 2014 release of the Thermotoga maritima MSB8 genome (AE000512.1), a revised annotation of the original release (Nelson et al., 1999). The sequence length and molecular weight were validated against those provided.
Pseudomonas aeruginosa Data
Outcomes in Additional file 1 (Madhavan et al., 2010) were matched to coding sequences provided by Constance Jeffrey.
Shine-Dalgarno-like mutagenesis Data
Folded protein is quantified by densitometry measurement (Schindelin et al., 2012; Schneider et al., 2012) of the relevant band in Figure 6 of (Fluman et al., 2014). Relative difference is calculated as is standard (Equation 1.2):
\[ \frac{\text{metric}_\text{mutant} - \text{metric}_\text{wildtype}}{\frac{1}{2}\left| \text{metric}_\text{mutant} - \text{metric}_\text{wildtype} \right|} \tag{1.2}\]
1.5.2 Details related to the calculation of sequence features
Transmembrane segment topology was predicted using Phobius Constrained for the training data and Phobius for all other datasets (Käll et al., 2004). We were able to obtain the Phobius code and integrate it directly into our feature calculation pipeline resulting in significantly faster speeds than any other option. Several features were obtained by averaging per-site metrics (e.g., per-residue RONN3.2 disorder predictions) in windows of a specified length. Windowed tAI metrics are calculated over all 30 base windows (not solely over 10 codon windows). Table 1.1 includes an in-depth description of each feature. Future work will explore contributions of elements outside the gene of interest, e.g., ribosomal binding site, linkers, tags.
1.5.3 Preparation for model learning
Calculated sequence features for the IMPs in the E. coli dataset as well as raw activity measurements, i.e., each 96-well plate, were loaded into R. As is best practice in using Support Vector Machines, each feature was “centered” and “scaled” where the mean value of a given feature was subtracted from each data point and then divided by the standard deviation of that feature using preprocess (Kuhn, 2008). As is standard practice, the resulting set was then culled for those features of near zero-variance, over 95% correlation (Pearson’s r), and linear dependence (nearZeroVar
, findCorrelation
, findLinearCombos
)(Kuhn, 2008). In particular this procedure removed extraneous degrees of freedom during the training process which carry little to no additional information with respect to the feature space and which may over represent certain redundant features. Features and outcomes for each list (“query”) were written into the SVMlight format using a modified svmlight.write
(Weihs et al., 2005).
The final features were calculated for each sequence in the test datasets, prepared for scoring by “centering” and “scaling” by the training set parameters via preprocess (Kuhn, 2008), and then written into SVMlight format again using a modified svmlight.write
.
1.5.4 Model selection, training, and evaluation using SVMrank
At the most basic level, our predictive model is a learned function that maps the parameter space (consisting of nucleotide and protein sequence features) to a response variable (expression level) through a set of governing weights (\(\vec{w}_1, \vec{w}_2, \ldots , \vec{w}_N\)). Depending on how the response variable is defined, these weights can be approximated using several different methods. As such, defining a response variable that is reflective of the available training data is key to selecting an appropriate learning algorithm.
The quantitative 96-well plate results (Daley et al., 2005) that comprise our training data do not offer an absolute expression metric valid over all plates—the top expressing proteins in one plate would not necessarily be the best expressing within another. As such, this problem is suited for preference-ranking methods. As a ranking problem, the response variable is the ordinal rank for each protein derived from its overexpression relative to the other members of the same plate of expression trials. In other words, the aim is to rank highly expressed proteins (based on numerous trials) at higher scores than lower expressed proteins by fitting against the order of expression outcomes from each constituent 96-well plate.
As the first work of this kind, the aim was to employ the simplest framework necessary taking in account the considerations above. The method chosen computes all valid pairwise classifications (i.e., within a single plate) transforming the original ranking problem into a binary classification problem. The algorithm outputs a score for each input by minimizing the number of swapped pairs thereby maximizing Kendall’s \(\tau\) (Kendall, 1938). For example, consider the following data generated via context \(A\): \((X_{A,1},\ Y_{A,1}), (X_{A,2},Y_{A,2})\) and \(B\): \((X_{B,1}, Y_{B,1}), (X_{B,2},Y_{B,2})\) where observed response follows as index \(i\), i.e., \(Y_{n} < Y_{n + 1}\). Binary classifier f (\(X_{i},\ X_{j})\) gives a score of 1 if an input pair matches its ordering criteria and \(- 1\) if not, i.e., \(Y_{i} < Y_{j}\):
\[ f\left( X_{A,1},X_{A,2} \right) = 1;f\left( X_{A,2},X_{A,1} \right) = - 1 \]
\[ f\left( X_{B,1},X_{B,2} \right) = 1;f\left( X_{B,2},X_{B,1} \right) = - 1 \]
\[ f\left( X_{A,1},X_{B,2} \right), f\left( X_{A,2},X_{B,1} \right) \text{ are invalid} \]
Free parameters describing \(f\) are calculated such that those calculated orderings \(f\left( X_{A,1} \right),f\left( X_{A,2} \right)\ldots;\ f\left( X_{B,1} \right),f\left( X_{B,2} \right)\ldots\) most closely agree (overall Kendall’s \(\tau\)) with the observed ordering \(\ Y_{n},\ Y_{n + 1},\ \ldots\). In this sense, \(f\) is a pairwise Learning to Rank method.
Within this class of models, a linear preference-ranking Support Vector Machine was employed (Joachims, 2002). To be clear, as an algorithm a preference-ranking SVM operates similarly to the canonical SVM binary classifier. In the traditional binary classification problem, a linear SVM seeks the maximally separating hyper-plane in the feature space between two classes, where class membership is determined by which side of the hyper-plane points reside. For some \(n\) linear separable training examples \(D = {\left\{ \ \left( x_{i} \right) \right|\ x_{i}\ \epsilon\ \mathbb{R}^{d}\}}^{n}\) and two classes \(y_{i}\ \epsilon\ \{ - 1,\ 1\}\), a linear SVM seeks a mapping from the d-dimensional feature space \(\mathbb{R}^{d} \rightarrow \{ - 1,\ 1\}\) by finding two maximally separated hyperplanes \(w\ \bullet x\ - b = 1\) and \(\ w\ \bullet x\ - b = - \ 1\) with constraints that \(w\ \bullet x_{i}\ - b \geq 1\ \)for all \(x_{i}\) with \(y_{i}\ \epsilon\ \{ 1\}\) and \(w\ \bullet x_{i}\ - b \leq \ - \ 1\) for all \(x_{i}\) with \(y_{i}\ \epsilon\ \{ - 1\}\). The feature weights correspond to the vector \(\vec{w}\), which is the vector perpendicular to the separating hyperplanes, and are computable in \(O(n\log{}n)\) implemented as part of the SVM^rank^ software package, though in \(O(n^2)\) (Tsochantaridis et al., 2005). See (Joachims, 2002) for an in-depth, technical discussion.
In a soft-margin SVM where training data is not linearly separable, a tradeoff between misclassified inputs and separation from the hyperplane must be specified. This parameter \(C\) was found by training models against raw data from Daley, Rapp, et al. with a grid of candidate \(C\) values (\(2^{n}\ \forall\ n\ \epsilon\ \lbrack - 5,\ \ 5\rbrack\)) and then evaluated against the raw “folded protein” measurements from Fluman, et al. The final model was chosen by selecting that with the lowest error from the process above (\(C = 2^5\)). To be clear, the final model is composed solely of a single weight for each feature; the tradeoff parameter \(C\) is only part of the training process.
Qualitatively, such a preference-ranking method constructs a model that ranks groups of proteins with higher expression level higher than other groups with lower expression value. In comparison to methods such as linear regression and binary classification, this approach is more robust and less affected by the inherent stochasticity of the training data.
1.5.5 Quantitative Assessment of Predictive Performance
In generating a predictive model, one aims to enrich for positive outcomes while ensuring they do not come at the cost of increased false positive diagnoses. This is formalized in Receiver Operating Characteristic (ROC) theory (for a primer see (Swets et al., 2000)), where the true positive rate is plotted against the false positive rate for all classification thresholds (score cutoffs in the ranked list). In this framework, the overall ability of the model to resolve positive from negative outcomes is evaluated by analyzing the Area Under a ROC curve (AUC) where AUCperfect=100% and AUCrandom=50% (percentage signs are omitted throughout the text and figures). All ROCs are calculated through pROC (Robin et al., 2011) using the analytic Delong method for AUC confidence intervals (DeLong et al., 1988). Bootstrapped AUC CIs (\(N = 10^6\)) were precise to 4 decimal places suggesting that analytic CIs are valid for the NYCOMPS dataset.
With several of our datasets, no definitive standard or clear-cut classification for positive expression exists. However, the aim is to show and test all reasonable classification thresholds of positive expression for each dataset in order to evaluate predictive performance as follows:
Training data
The outcomes are quantitative (activity level), so each ROC is calculated by normalizing within each dataset to the standard well subject to the discussion in 4a above (LepB for PhoA, and InvLepB for GFP) (examples in Figure 1.1D) for each possible threshold, i.e., each normalized expression value with each AUC plotted in Figure 1.1E. 95% confidence intervals of Spearman’s \(\rho\) are given by 106 iterations of a bias-corrected and accelerated (BCa) bootstrap of the data (Figure 1.1A,C) (Canty and Ripley, 2015).
Large-scale
ROCs were calculated for each of the expression classes (Figure 1.2E). Regardless of the split, predictive performance is noted. The binwidth for the histogram was determined using the Freedman-Diaconis rule(Freedman and Diaconis, 1981), and scores outside the plotted range comprising <0.6% of the density were implicitly hidden.
Small-scale
Classes can be defined in many different ways. To be principled about the matter, ROCs for each possible cutoff are presented based on definitions from each publication (Figure 1.3C,E,G; Figure 1.8B,D,F). See Section 1.5.1 for any necessary details about outcome classifications for each dataset.
1.5.6 Feature Weights
Weights for the learned SVM are pulled directly from the model file produced by SVMlight and are given in Table 1.1.
1.5.7 Availability
All analysis is documented in a series of R notebooks (Xie, 2014) available openly at github.com/clemlab/ml-ecoli-IMProve. These notebooks provide fully executable instructions for the reproduction of the analyses and the generation of figures and statistics in this study. The IMProve model is available as a web service at clemonslab.caltech.edu.
1.6 Acknowledgements
We thank Daniel Daley and Thomas Miller’s group for discussion, Yaser Abu-Mostafa and Yisong Yue for guidance regarding machine learning, Niles Pierce for providing NUPACK source code (Zadeh et al., 2011), Welison Floriano and Naveed Near-Ansari for maintaining local computing resources, and Samuel Schulte for suggesting the model’s name. We thank Michiel Niesen, Stephen Marshall, Thomas Miller, Reid van Lehn, James Bowie, and Tom Rapoport for comments on the manuscript. Models and analyses are possible thanks to raw experimental data provided by Daniel Daley and Mikaela Rapp (Daley et al., 2005); Nir Fluman (Fluman et al., 2014); Edda Kloppmann, Brian Kloss, and Marco Punta from NYCOMPS (Love et al., 2010; Punta et al., 2009); Pikyee Ma (Ma et al., 2013); Renaud Wagner (Lundstrom et al., 2006); Florent Bernaudat (Bernaudat et al., 2011), and Constance Jeffrey (Madhavan et al., 2010).
We acknowledge funding from an NIH Pioneer Award to WMC (5DP1GM105385); a Benjamin M. Rosen graduate fellowship, a NIH/NRSA training grant (5T32GM07616), and a NSF Graduate Research fellowship to SMS; and an Arthur A. Noyes Summer Undergraduate Research Fellowship to NJ. Computational time was provided by Stephen Mayo and Douglas Rees. This material is based upon work supported by the National Science Foundation Graduate Research Fellowship Program under Grant No. 1144469. Any opinions, findings, and conclusions or recommendations expressed in this material are those of the authors and do not necessarily reflect the views of the National Science Foundation. This work used the Extreme Science and Engineering Discovery Environment (XSEDE), which is supported by National Science Foundation grant number ACI-1053575 (Towns et al., 2014).
1.7 Supplementary Figures
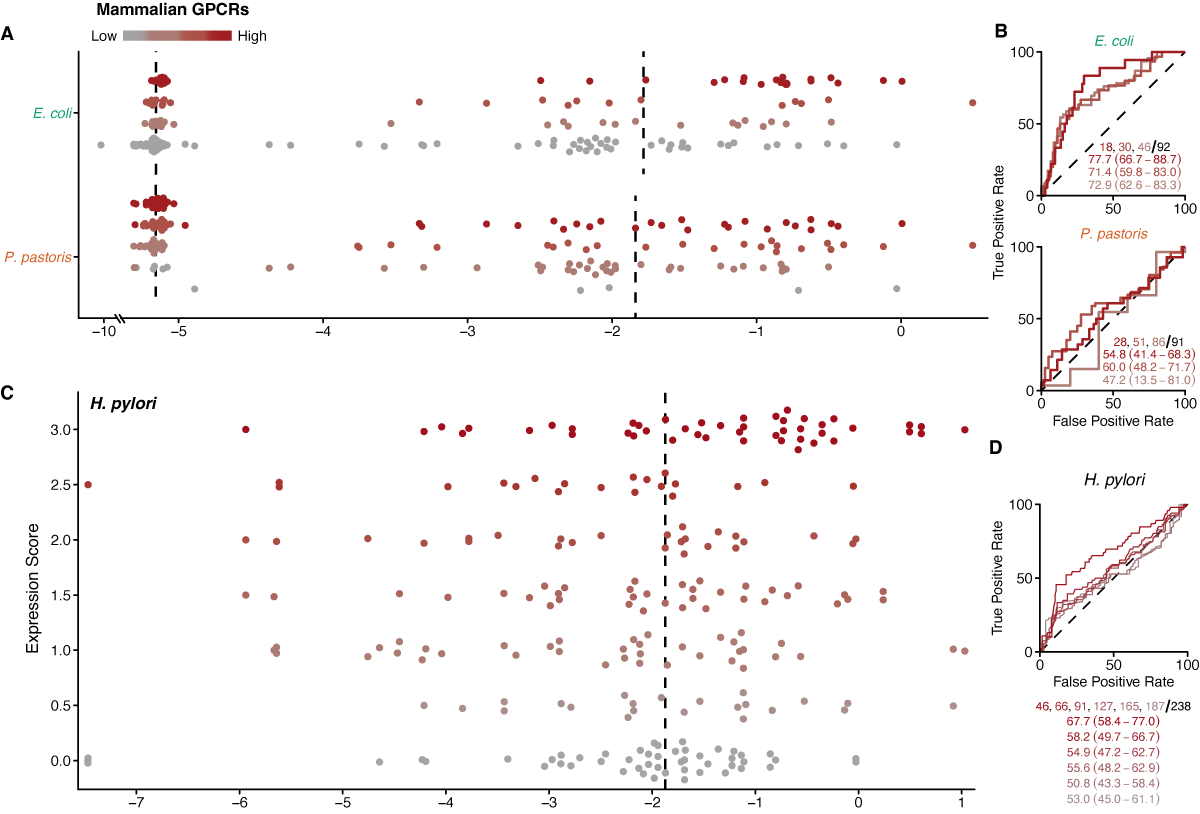
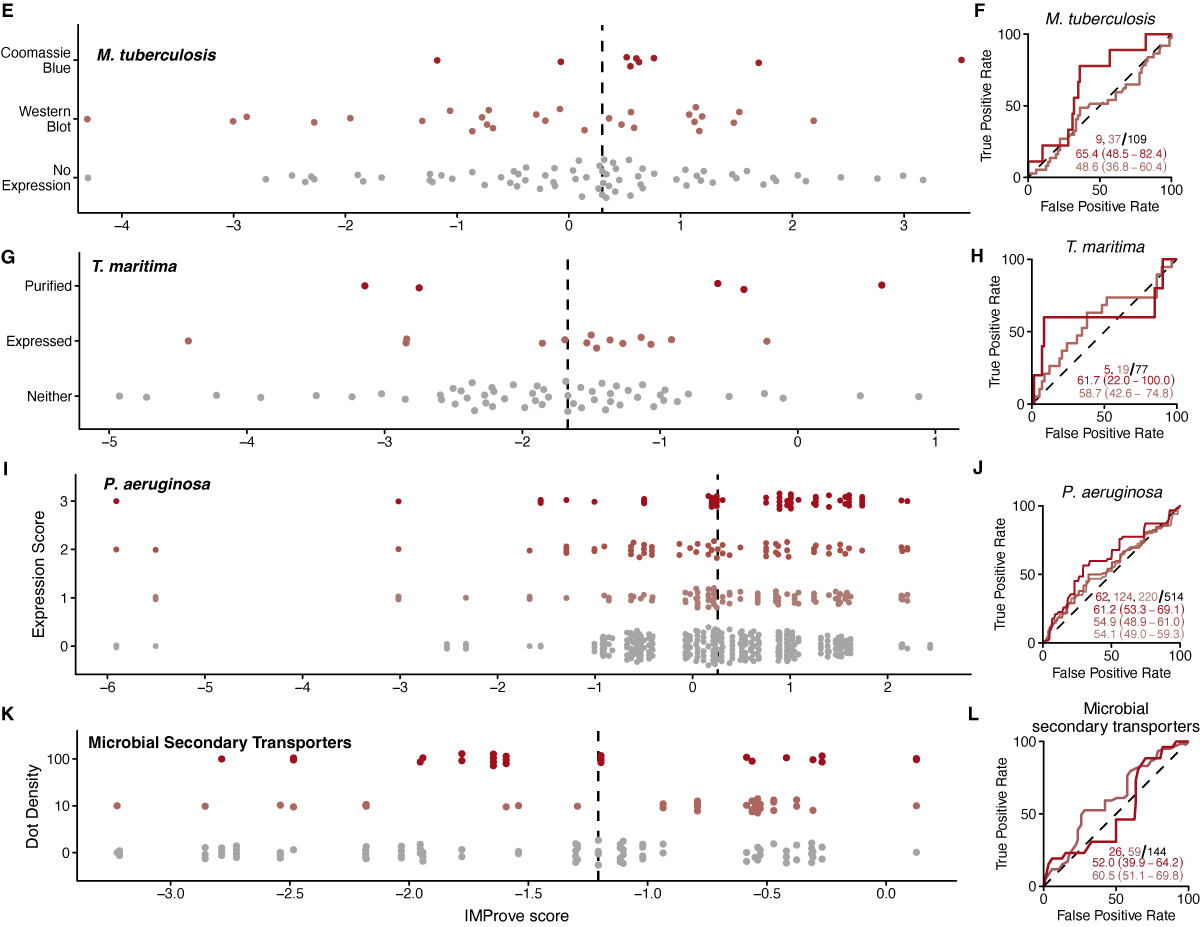
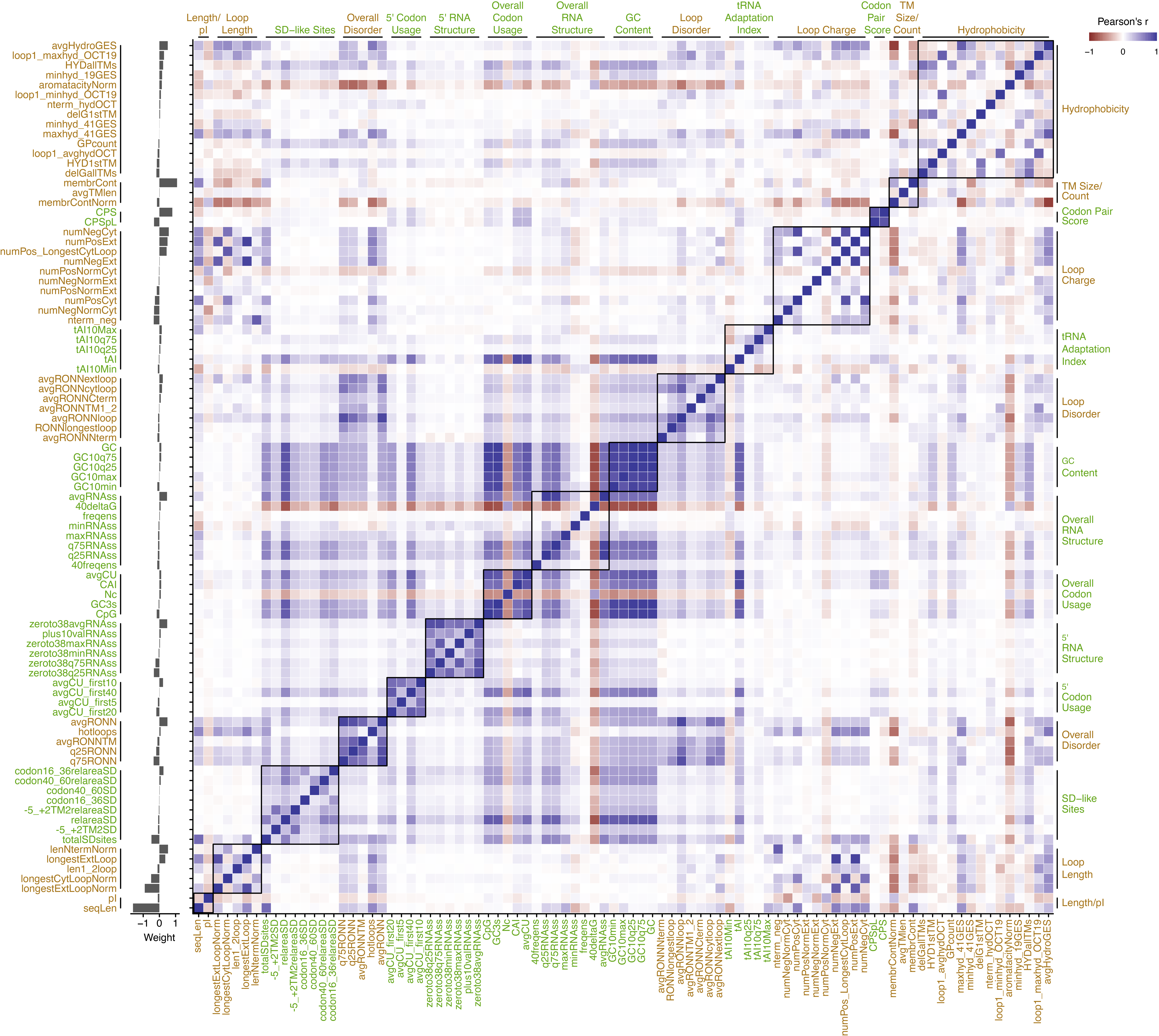
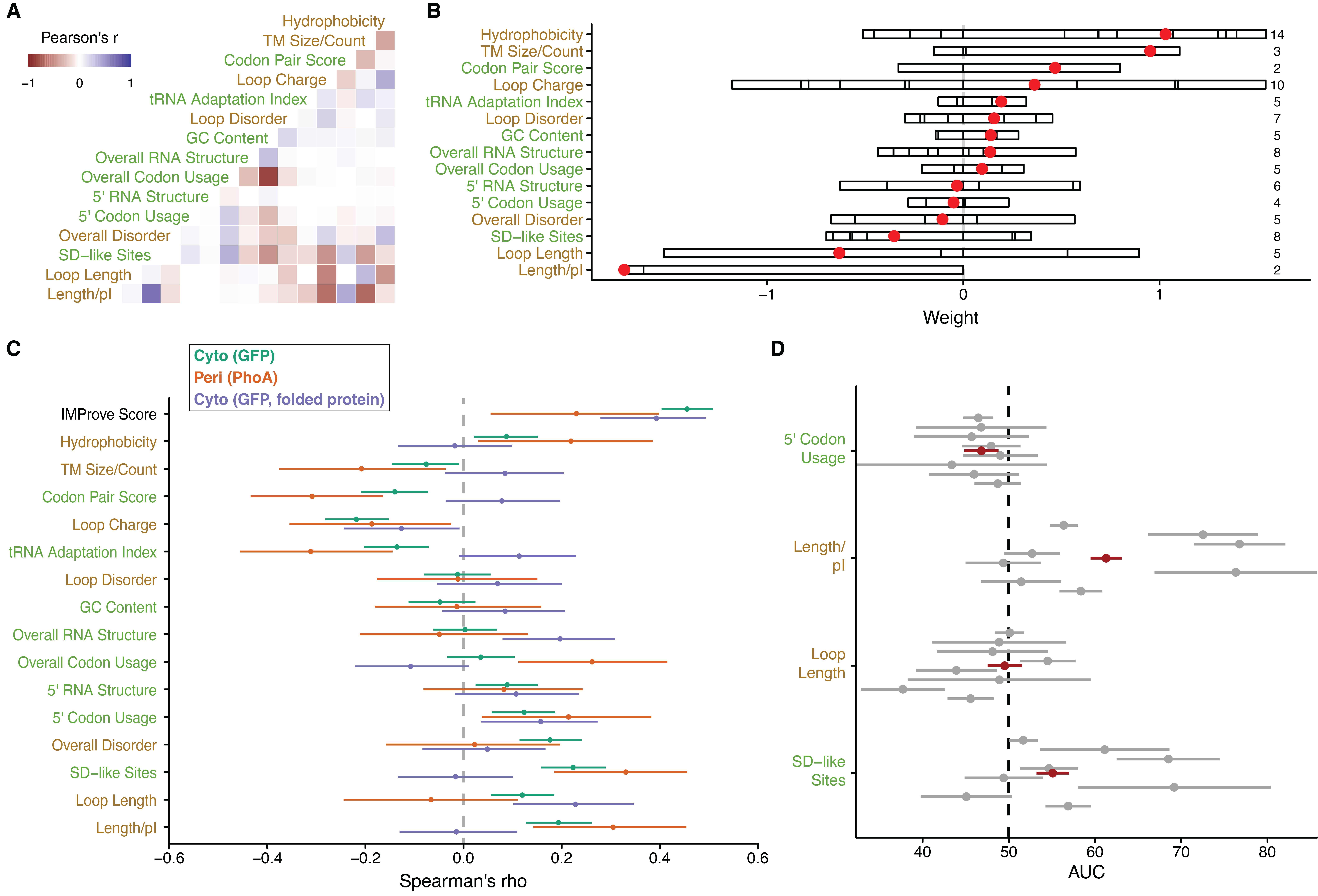
1.8 Supplementary Tables
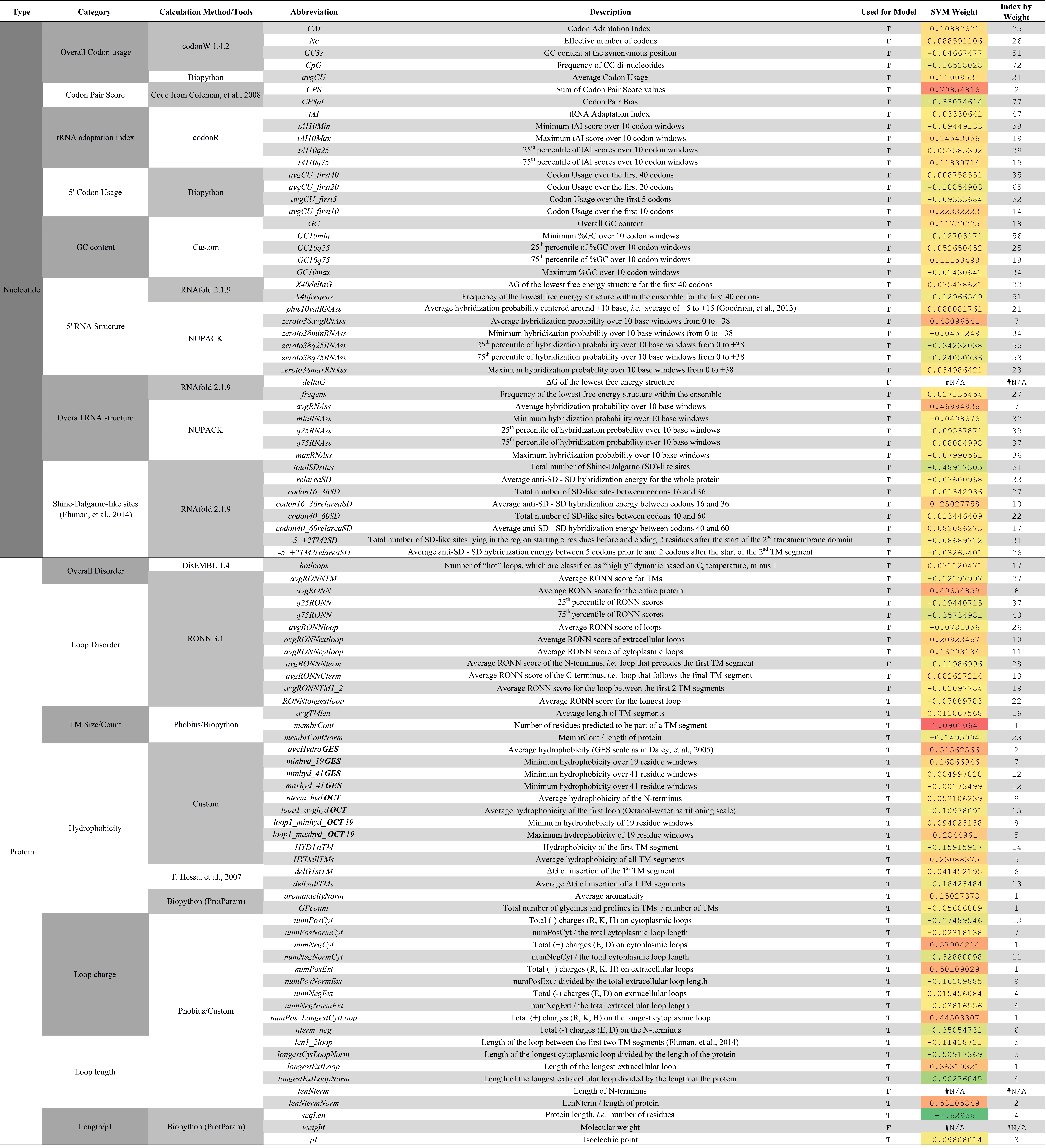
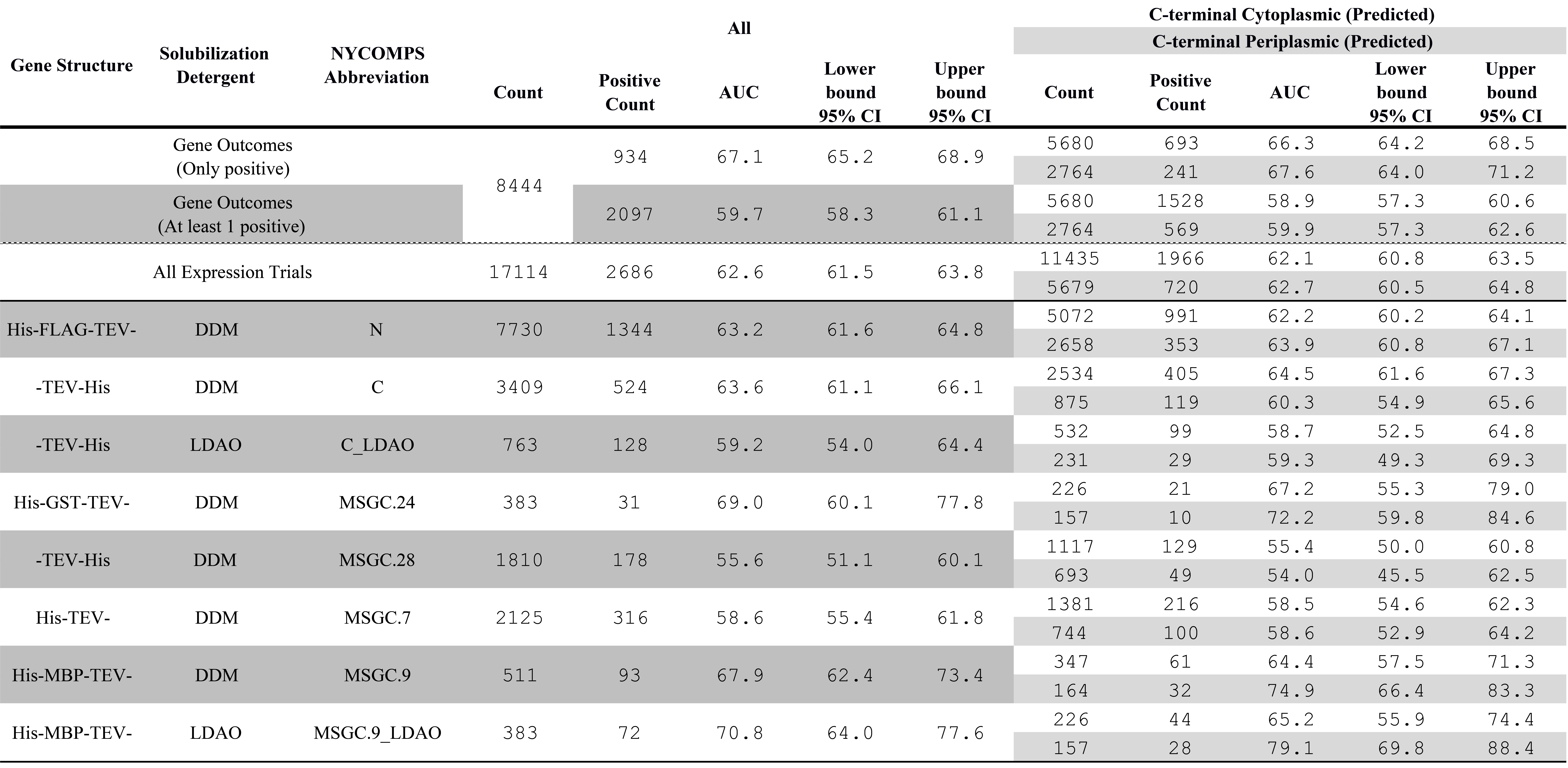